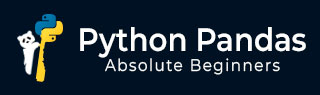
- Python Pandas - Home
- Python Pandas - Introduction
- Python Pandas - Environment Setup
- Python Pandas - Basics
- Python Pandas - Introduction to Data Structures
- Python Pandas - Index Objects
- Python Pandas - Panel
- Python Pandas - Basic Functionality
- Python Pandas - Indexing & Selecting Data
- Python Pandas - Series
- Python Pandas - Series
- Python Pandas - Slicing a Series Object
- Python Pandas - Attributes of a Series Object
- Python Pandas - Arithmetic Operations on Series Object
- Python Pandas - Converting Series to Other Objects
- Python Pandas - DataFrame
- Python Pandas - DataFrame
- Python Pandas - Accessing DataFrame
- Python Pandas - Slicing a DataFrame Object
- Python Pandas - Modifying DataFrame
- Python Pandas - Removing Rows from a DataFrame
- Python Pandas - Arithmetic Operations on DataFrame
- Python Pandas - IO Tools
- Python Pandas - IO Tools
- Python Pandas - Working with CSV Format
- Python Pandas - Reading & Writing JSON Files
- Python Pandas - Reading Data from an Excel File
- Python Pandas - Writing Data to Excel Files
- Python Pandas - Working with HTML Data
- Python Pandas - Clipboard
- Python Pandas - Working with HDF5 Format
- Python Pandas - Comparison with SQL
- Python Pandas - Data Handling
- Python Pandas - Sorting
- Python Pandas - Reindexing
- Python Pandas - Iteration
- Python Pandas - Concatenation
- Python Pandas - Statistical Functions
- Python Pandas - Descriptive Statistics
- Python Pandas - Working with Text Data
- Python Pandas - Function Application
- Python Pandas - Options & Customization
- Python Pandas - Window Functions
- Python Pandas - Aggregations
- Python Pandas - Merging/Joining
- Python Pandas - MultiIndex
- Python Pandas - Basics of MultiIndex
- Python Pandas - Indexing with MultiIndex
- Python Pandas - Advanced Reindexing with MultiIndex
- Python Pandas - Renaming MultiIndex Labels
- Python Pandas - Sorting a MultiIndex
- Python Pandas - Binary Operations
- Python Pandas - Binary Comparison Operations
- Python Pandas - Boolean Indexing
- Python Pandas - Boolean Masking
- Python Pandas - Data Reshaping & Pivoting
- Python Pandas - Pivoting
- Python Pandas - Stacking & Unstacking
- Python Pandas - Melting
- Python Pandas - Computing Dummy Variables
- Python Pandas - Categorical Data
- Python Pandas - Categorical Data
- Python Pandas - Ordering & Sorting Categorical Data
- Python Pandas - Comparing Categorical Data
- Python Pandas - Handling Missing Data
- Python Pandas - Missing Data
- Python Pandas - Filling Missing Data
- Python Pandas - Interpolation of Missing Values
- Python Pandas - Dropping Missing Data
- Python Pandas - Calculations with Missing Data
- Python Pandas - Handling Duplicates
- Python Pandas - Duplicated Data
- Python Pandas - Counting & Retrieving Unique Elements
- Python Pandas - Duplicated Labels
- Python Pandas - Grouping & Aggregation
- Python Pandas - GroupBy
- Python Pandas - Time-series Data
- Python Pandas - Date Functionality
- Python Pandas - Timedelta
- Python Pandas - Sparse Data Structures
- Python Pandas - Sparse Data
- Python Pandas - Visualization
- Python Pandas - Visualization
- Python Pandas - Additional Concepts
- Python Pandas - Caveats & Gotchas
Python Pandas to_json() Method
The to_json() method in Python's Pandas library is used to convert a Pandas DataFrame or Series into a JSON string or save it to a JSON file. This method provides a number of options to control the format of the resulting JSON string, such as the orientation of JSON data and the formatting of date and time. This method can be useful for working with APIs, exporting data, or storing data in a portable and structured format. It can also handle various cases like NaN values, datetime objects, and custom unsupported data types.
JSON (JavaScript Object Notation) is a popular lightweight data-interchange format for storing structured information. It uses plain-text formatting, where each element is represented in a hierarchical structure, making it easy to read and process. JSON files have the .json extension and are commonly used in web applications, APIs, and data pipelines.
Syntax
Following is the syntax of the Python to_json() method −
DataFrame.to_json(path_or_buf=None, *, orient=None, date_format=None, double_precision=10, force_ascii=True, date_unit='ms', default_handler=None, lines=False, compression='infer', index=None, indent=None, storage_options=None, mode='w')
Note: When using the to_json() method on a Pandas Series object, you should call it as Series.to_json().
Parameters
The to_json() method accepts the following parameters −
path_or_buf: A string that specifies the location for the output JSON file. It can be a file path or a buffer. If set to None, the result is returned as JSON string.
orient: Defines the format of the JSON string. Possible values are: 'split', 'records', 'index', 'columns', 'values', 'table'. The default value is 'columns' for a DataFrame, and 'index' for a Series.
date_format: Specifies the format for dates. It can be 'epoch' (default) or 'iso' for ISO8601 date format, this option is default for table orientation.
double_precision: Sets the number of decimal places for floating-point values. The default is 10.
force_ascii: Forces all strings in the output to be ASCII-encoded. Default is True.
date_unit: Specifies the time unit for encoding dates, such as 'ms' (milliseconds), 's' (seconds), etc. Default is 'ms'.
default_handler: A callable function that handles objects which cannot be automatically converted to JSON format. It returns a serializable object.
lines: If True and orient is 'records', and the output is in a line-delimited JSON format. Default is False.
compression: Defines compression for the output file. Accepted values include 'infer', 'gzip', 'bz2', 'zip', and others.
index: Specifies whether the index should be included in the output JSON. Default is None.
indent: Sets the number of spaces used for indentation in the JSON output. Default is None (no indentation).
storage_options: Allows passing additional options for specific storage backends like HTTP or cloud storage (e.g., 's3://', and 'gcs://').
mode: Defines the IO mode. Accepted values are 'w' (write) and 'a' (append). Default is 'w'.
Return Value
The to_json() method returns a JSON string if the path_or_buf parameter is set to None. If a file or buffer is provided, it writes the JSON string to the given location and returns None.
Example: Converting DataFrame to JSON String
Here is a basic example of using the to_json() method to convert a DataFrame into a JSON string with the default 'columns' orientation.
import pandas as pd # Create a DataFrame df = pd.DataFrame( [["a", "b"], ["c", "d"]], index=["row_1", "row_2"], columns=["col_1", "col_2"], ) # Convert to JSON result = df.to_json() # Print the resulting JSON print(result)
When we run the above program, it produces the following result:
{"col_1":{"row_1":"a","row_2":"c"},"col_2":{"row_1":"b","row_2":"d"}}
Example: DataFrame to JSON String with 'records' Orientation
Now let's use the 'records' orientation option of the DataFrame.to_json() method, which will result in a list of dictionaries where each dictionary represents a row of the DataFrame.
import pandas as pd # Create a DataFrame df = pd.DataFrame( [["a", "b"], ["c", "d"]], index=["row_1", "row_2"], columns=["col_1", "col_2"], ) # Convert to JSON with 'records' orientation result = df.to_json(orient="records") # Print the resulting JSON print(result)
Following is an output of the above code −
[{"col_1":"a","col_2":"b"},{"col_1":"c","col_2":"d"}]
Example: Exporting DataFrame to JSON with Table Schema
In this example, we will use the 'table' orientation, which includes additional schema information along with the data.
import pandas as pd # Create a DataFrame df = pd.DataFrame( [["a", "b"], ["c", "d"]], index=["row_1", "row_2"], columns=["col_1", "col_2"], ) # Convert to JSON with 'table' orientation result = df.to_json(orient="table") # Print the resulting JSON print(result)
Output of the above code is as follows:
{"schema":{"fields":[{"name":"index","type":"string"},{"name":"col_1","type":"string"},{"name":"col_2","type":"string"}],"primaryKey":["index"],"pandas_version":"1.4.0"},"data":[{"index":"row_1","col_1":"a","col_2":"b"},{"index":"row_2","col_1":"c","col_2":"d"}]}
Example: Saving DataFrame to JSON File
In this example, we will save the Pandas DataFrame into a JSON file using the DataFrame.to_json() method, by specifying the string representing the file path.
import pandas as pd # Create a DataFrame df = pd.DataFrame( [["a", "b"], ["c", "d"]], index=["row_1", "row_2"], columns=["col_1", "col_2"], ) # Convert to JSON file df.to_json('dataframe_to_json_data.json') print("DataFrame has been saved to 'dataframe_to_json_data.json'")
Output of the above code is as follows −
DataFrame has been saved to 'dataframe_to_json_data.json'
Example: Customizing JSON Indentation
This example demonstrates how to use the to_json() method to convert a DataFrame into a JSON string with custom indentation.
import pandas as pd # Create a DataFrame df = pd.DataFrame( [["a", "b"], ["c", "d"]], index=["row_1", "row_2"], columns=["col_1", "col_2"], ) # Convert to JSON with Custom Indentation result = df.to_json(indent=3) # Print the resulting JSON string print(result)
When we run the above program, it produces the following result:
{ "col_1":{ "row_1":"a", "row_2":"c" }, "col_2":{ "row_1":"b", "row_2":"d" } }