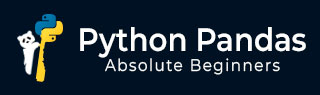
- Python Pandas - Home
- Python Pandas - Introduction
- Python Pandas - Environment Setup
- Python Pandas - Basics
- Python Pandas - Introduction to Data Structures
- Python Pandas - Index Objects
- Python Pandas - Panel
- Python Pandas - Basic Functionality
- Python Pandas - Indexing & Selecting Data
- Python Pandas - Series
- Python Pandas - Series
- Python Pandas - Slicing a Series Object
- Python Pandas - Attributes of a Series Object
- Python Pandas - Arithmetic Operations on Series Object
- Python Pandas - Converting Series to Other Objects
- Python Pandas - DataFrame
- Python Pandas - DataFrame
- Python Pandas - Accessing DataFrame
- Python Pandas - Slicing a DataFrame Object
- Python Pandas - Modifying DataFrame
- Python Pandas - Removing Rows from a DataFrame
- Python Pandas - Arithmetic Operations on DataFrame
- Python Pandas - IO Tools
- Python Pandas - IO Tools
- Python Pandas - Working with CSV Format
- Python Pandas - Reading & Writing JSON Files
- Python Pandas - Reading Data from an Excel File
- Python Pandas - Writing Data to Excel Files
- Python Pandas - Working with HTML Data
- Python Pandas - Clipboard
- Python Pandas - Working with HDF5 Format
- Python Pandas - Comparison with SQL
- Python Pandas - Data Handling
- Python Pandas - Sorting
- Python Pandas - Reindexing
- Python Pandas - Iteration
- Python Pandas - Concatenation
- Python Pandas - Statistical Functions
- Python Pandas - Descriptive Statistics
- Python Pandas - Working with Text Data
- Python Pandas - Function Application
- Python Pandas - Options & Customization
- Python Pandas - Window Functions
- Python Pandas - Aggregations
- Python Pandas - Merging/Joining
- Python Pandas - MultiIndex
- Python Pandas - Basics of MultiIndex
- Python Pandas - Indexing with MultiIndex
- Python Pandas - Advanced Reindexing with MultiIndex
- Python Pandas - Renaming MultiIndex Labels
- Python Pandas - Sorting a MultiIndex
- Python Pandas - Binary Operations
- Python Pandas - Binary Comparison Operations
- Python Pandas - Boolean Indexing
- Python Pandas - Boolean Masking
- Python Pandas - Data Reshaping & Pivoting
- Python Pandas - Pivoting
- Python Pandas - Stacking & Unstacking
- Python Pandas - Melting
- Python Pandas - Computing Dummy Variables
- Python Pandas - Categorical Data
- Python Pandas - Categorical Data
- Python Pandas - Ordering & Sorting Categorical Data
- Python Pandas - Comparing Categorical Data
- Python Pandas - Handling Missing Data
- Python Pandas - Missing Data
- Python Pandas - Filling Missing Data
- Python Pandas - Interpolation of Missing Values
- Python Pandas - Dropping Missing Data
- Python Pandas - Calculations with Missing Data
- Python Pandas - Handling Duplicates
- Python Pandas - Duplicated Data
- Python Pandas - Counting & Retrieving Unique Elements
- Python Pandas - Duplicated Labels
- Python Pandas - Grouping & Aggregation
- Python Pandas - GroupBy
- Python Pandas - Time-series Data
- Python Pandas - Date Functionality
- Python Pandas - Timedelta
- Python Pandas - Sparse Data Structures
- Python Pandas - Sparse Data
- Python Pandas - Visualization
- Python Pandas - Visualization
- Python Pandas - Additional Concepts
- Python Pandas - Caveats & Gotchas
Pandas Cheatsheet
The Pandas cheatsheet provides a fundamental reference to all the core concepts of pandas. This powerful library in Python is used for data manipulation, analysis, and handling structured data with ease. Whether you're working with large datasets, performing data cleaning, or analyzing trends, this cheat sheet will help you navigate Pandas easily. Go through the cheatsheet and learn the Python pandas library.
- Introduction to Pandas
- Installing Pandas
- Creating DataFrames
- Creating Series
- Reading Data
- Writing Data
- Selecting Columns
- Selecting Rows
- Filtering Data
- Boolean Indexing
- Querying Data
- Handling Missing Values
- Changing Data Types
- Renaming Columns
- Duplicates
- Replacing Values
- Sorting Data
- GroupBy
- Pivot Tables
- Apply Functions
- Merging and Joining
- Summary Statistics
- Value Counts
- Correlation
- Cumulative Functions
- MultiIndex
- Time Series Analysis
- Working with JSON
- Visualization
1. Introduction to Pandas
In the introduction, Pandas is a popular open-source library in Python for data analysis. It provides data structures and functions to processes large datasets which includes tabular data such as spreadsheets and SQL tables. Here, we will learn how to import the pandas library.
import pandas as pd
2. Installing Pandas
To install Pandas on the system, use the following command −
pip install pandas
3. Creating DataFrames
The dataframe can be created using lists, dictionaries, and external data sources.
# Creating a DataFrame from a dictionary import pandas as pd inp_data = {"Name": ["Ravi", "Faran"], "Age": [25, 30]} df = pd.DataFrame(inp_data) print(df)
4. Creating Series
In Pandas, the series is like a column in the table. You can create the pandas series using a list or NumPy arrays.
import pandas as pd s = pd.Series([10, 20, 30, 40])
5. Reading Data
There are four methods to read data in Pandas − CSV, Excel, JSON, and SQL files.
# Reading a CSV file df = pd.read_csv("data.csv")
6. Writing Data
To write the pandas dataframe in a CSV file, the user needs Dataframe.to_csv().
# Writing a DataFrame to a CSV file df.to_csv("output.csv", index=False)
7. Selecting Columns
To select the specific column from dataframe −
# Selecting a single column df["Name"]
8. Selecting Rows
To retrieve specific rows using index selection and slicing, Pandas provides the head() and tail() methods. The head() method returns the first few rows of the DataFrame, while the tail() method retrieves the last few rows.
df.head(5)
Or,
df.tail(5)
9. Filtering Data
Filtering data in pandas means it applies some conditions based on certain rows and columns.
# Filtering rows where Age > 25 df[df["Age"] > 25]
10. Boolean Indexing
In pandas, boolean indexing means the process of filtering data using a boolean array.
mask = df["Age"] > 25 df[mask]
11. Querying Data
In Pandas, querying data filters the dataframe by passing the condition as a string that returns matching rows. You can use the query() method.
df.query("Age > 25")
12. Handling Missing Values
To handle the missing values in Pandas, use the methods like dropna() and fillna(). Below is the implementation −
df.fillna(0, inplace=True)
Or,
import pandas as pd # Creating a DataFrame with missing values data = {"Name": ["Vivek", "Faran", None, "Revathi"], "Age": [25, None, 30, 35]} df = pd.DataFrame(data) # Dropping rows with missing values df_result = df.dropna() print(df_result)
13. Changing Data Types
To convert the data types in Python use the method astype(). This ensures the proper formatting.
df["Age"] = df["Age"].astype(int)
14. Renaming Columns
The easier way to rename the columns in Pandas, use the method rename(). The following syntax is given below −
df.rename(columns={"old_name": "new_name"}, inplace=True)
15. Duplicates
To remove the duplicates from the rows, use the method drop_duplicates().
df.drop_duplicates(inplace=True)
16. Replacing Values
The term "replacing" is also known as "removing". To remove the specific values in a dataframe, use the method replace().
df["column_name"].replace({"old_value": "new_value"}, inplace=True)
17. Sorting Data
In Python, Pandas is a popular library that provides a built-in method called sort_values(). This method allows users to sort the values of a DataFrame or Series in ascending or descending order.
import pandas as pd data = {'Name': ['Alex', 'John', 'Sunny', 'Usha'], 'Id': [2115, 6330, 8135, 4110], 'Score': [85, 90, 95, 80]} df = pd.DataFrame(data) # Sorting by 'Id' in ascending order sorted_df = df.sort_values(by='Id') print(sorted_df)
18. GroupBy
GroupBy is used to split the data into groups based on some criteria and then apply a function to each group. Thus, this helps in data summarization and analyzing.
# Grouping by 'Gender' and calculating the mean age df.groupby('Gender')['Age'].mean()
19. Pivot Tables
In Pandas, the use of pivot tables is to summarize the data that allows users to aggregate data across multiple dimensions.
df.pivot_table(values='Age', index='Gender', columns='City', aggfunc='mean')
20. Apply Functions
In pandas, the apply() function is used to apply a function along the axis of a DataFrame or Series.
df.apply(lambda x: x.max() - x.min())
21. Merging and Joining
In Pandas, the concept of merging and joining allows users to combine multiple dataframes based on shared columns or indexes.
# Merging two DataFrames on a common column 'ID' df1.merge(df2, on='ID')
Or,
df1.join(df2, on='column_name', how='inner')
Explanation of join() parameters −
- on: Specifies the column or index to join on.
- how: This determine the type of join used for the dataset.
22. Summary Statistics
Summary statistics help in understanding the distribution and key properties of the dataset. Methods like 'mean()', 'median()', and 'std()' provide insights of the data from the given datasets.
# Getting summary statistics df.describe()
23. Value Counts
The value_counts() method is used to get the frequency of unique values in a column.
df['col_name'].value_counts()
24. Correlation
Correlation means the relationship between two variables. The corr() method calculates the correlation coefficient between columns in a DataFrame.
df.corr()
25. Cumulative Functions
In Pandas, cumulative functions are those functions that add up or multiply values sequentially over time. You can use methods like cumsum() and cumprod().
df['Age'].cumsum()
26. MultiIndex
The MultiIndex is a very simple concept that adds multiple levels of indexing in a DataFrame. So, it is possible to handle the complex data structure.
arrays = [['A', 'A', 'B', 'B'], [1, 2, 1, 2]] index = pd.MultiIndex.from_arrays(arrays, names=('Letter', 'Number')) df_multi = pd.DataFrame({'Value': [10, 20, 30, 40]}, index=index)
27. Time Series Analysis
Time series analysis works with time-indexed data. Pandas provides functionality to handle time series data by containing date parsing and resampling.
# Converting a column to datetime format df['Date'] = pd.to_datetime(df['Date']) # Resampling data by month df.resample('M').mean()
28. Working with JSON
JSON (JavaScript Object Notation) is a popular data format. In pandas, we have two ways to implement JSON −
- read_json() − It read the JSON data into a dataframe.
- to_json() − It convert dataframes into JSON format.
# read JSON data into a DataFrame df = pd.read_json('data.json') # convert DataFrame into JSON df.to_json('output.json')
29. Visualization
Data visualization is key to understanding patterns and insights. Pandas integrates with libraries like Matplotlib and Seaborn to create various plots from DataFrames.
# Plotting a line graph using Pandas df['Age'].plot(kind='line') # Plotting a histogram df['Age'].plot(kind='hist', bins=10)