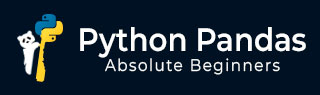
- Python Pandas - Home
- Python Pandas - Introduction
- Python Pandas - Environment Setup
- Python Pandas - Basics
- Python Pandas - Introduction to Data Structures
- Python Pandas - Index Objects
- Python Pandas - Panel
- Python Pandas - Basic Functionality
- Python Pandas - Indexing & Selecting Data
- Python Pandas - Series
- Python Pandas - Series
- Python Pandas - Slicing a Series Object
- Python Pandas - Attributes of a Series Object
- Python Pandas - Arithmetic Operations on Series Object
- Python Pandas - Converting Series to Other Objects
- Python Pandas - DataFrame
- Python Pandas - DataFrame
- Python Pandas - Accessing DataFrame
- Python Pandas - Slicing a DataFrame Object
- Python Pandas - Modifying DataFrame
- Python Pandas - Removing Rows from a DataFrame
- Python Pandas - Arithmetic Operations on DataFrame
- Python Pandas - IO Tools
- Python Pandas - IO Tools
- Python Pandas - Working with CSV Format
- Python Pandas - Reading & Writing JSON Files
- Python Pandas - Reading Data from an Excel File
- Python Pandas - Writing Data to Excel Files
- Python Pandas - Working with HTML Data
- Python Pandas - Clipboard
- Python Pandas - Working with HDF5 Format
- Python Pandas - Comparison with SQL
- Python Pandas - Data Handling
- Python Pandas - Sorting
- Python Pandas - Reindexing
- Python Pandas - Iteration
- Python Pandas - Concatenation
- Python Pandas - Statistical Functions
- Python Pandas - Descriptive Statistics
- Python Pandas - Working with Text Data
- Python Pandas - Function Application
- Python Pandas - Options & Customization
- Python Pandas - Window Functions
- Python Pandas - Aggregations
- Python Pandas - Merging/Joining
- Python Pandas - MultiIndex
- Python Pandas - Basics of MultiIndex
- Python Pandas - Indexing with MultiIndex
- Python Pandas - Advanced Reindexing with MultiIndex
- Python Pandas - Renaming MultiIndex Labels
- Python Pandas - Sorting a MultiIndex
- Python Pandas - Binary Operations
- Python Pandas - Binary Comparison Operations
- Python Pandas - Boolean Indexing
- Python Pandas - Boolean Masking
- Python Pandas - Data Reshaping & Pivoting
- Python Pandas - Pivoting
- Python Pandas - Stacking & Unstacking
- Python Pandas - Melting
- Python Pandas - Computing Dummy Variables
- Python Pandas - Categorical Data
- Python Pandas - Categorical Data
- Python Pandas - Ordering & Sorting Categorical Data
- Python Pandas - Comparing Categorical Data
- Python Pandas - Handling Missing Data
- Python Pandas - Missing Data
- Python Pandas - Filling Missing Data
- Python Pandas - Interpolation of Missing Values
- Python Pandas - Dropping Missing Data
- Python Pandas - Calculations with Missing Data
- Python Pandas - Handling Duplicates
- Python Pandas - Duplicated Data
- Python Pandas - Counting & Retrieving Unique Elements
- Python Pandas - Duplicated Labels
- Python Pandas - Grouping & Aggregation
- Python Pandas - GroupBy
- Python Pandas - Time-series Data
- Python Pandas - Date Functionality
- Python Pandas - Timedelta
- Python Pandas - Sparse Data Structures
- Python Pandas - Sparse Data
- Python Pandas - Visualization
- Python Pandas - Visualization
- Python Pandas - Additional Concepts
- Python Pandas - Caveats & Gotchas
Python Pandas - Stacking and Unstacking
Stacking and unstacking in Pandas are the useful techniques for reshaping DataFrames to extract more information in different ways. It works efficiently with multi-level indices also. Whether it's compressing columns into row levels or expanding rows into columns, these operations are crucial for handling complex datasets.
The Pandas library provides two main methods for these operations −
stack(): Used for Stacking, converts columns into row indices, creating a long-format DataFrame.
unstack(): For Unstacking, which moves an index level back to columns, converting long-format data into a wide format.
In this tutorial, we will learn about stacking and unstacking techniques in Pandas along with practical examples, including handling missing data.
Stacking in Pandas
Stacking in Pandas is a process of compressing a DataFrame columns into rows. The DataFrame.stack() method in Pandas is used for stacking the levels from column to index. This method pivots a level of column labels (possibly hierarchical) into row labels, and returns a new DataFrame or Series with a multi-level index.
Example
Following example uses the df.stack() method for pivoting the columns into the row index.
import pandas as pd import numpy as np # Create MultiIndex tuples = [["x", "x", "y", "y", "", "f", "z", "z"],["1", "2", "1", "2", "1", "2", "1", "2"]] index = pd.MultiIndex.from_arrays(tuples, names=["first", "second"]) # Create a DataFrame df = pd.DataFrame(np.random.randn(8, 2), index=index, columns=["A", "B"]) # Display the input DataFrame print('Input DataFrame:\n', df) # Stack columns stacked = df.stack() print('Output Reshaped DataFrame:\n', stacked)
Following is the output of the above code −
Input DataFrame:
A | B | ||
---|---|---|---|
first | second | ||
x | 1 | 0.596485 | -1.356041 |
2 | -1.091407 | 0.246216 | |
y | 1 | 0.499328 | -1.346817 |
2 | -0.893557 | 0.014678 | |
1 | -0.059916 | 0.106597 | |
f | 2 | -0.315096 | -0.950424 |
z | 1 | 1.050350 | -1.744569 |
2 | -0.255863 | 0.539803 |
first | second | ||
---|---|---|---|
x | 1 | A | 0.596485 |
B | -1.356041 | ||
2 | A | -1.091407 | |
B | 0.246216 | ||
y | 1 | A | 0.499328 |
B | -1.346817 | ||
2 | A | -0.893557 | |
B | 0.014678 | ||
1 | A | -0.059916 | |
B | 0.106597 | ||
f | 2 | A | -0.315096 |
B | -0.950424 | ||
z | 1 | A | 1.050350 |
B | -1.744569 | ||
2 | A | -0.255863 | |
B | 0.539803 |
Here, the stack() method pivots the columns A and B into the index, compressing the DataFrame into a long format.
Unstacking in Pandas
Unstacking reverses the stacking operation by moving the row index level back to the columns. The Pandas DataFrame.unstack() method is used to pivot a level of the row index to become a column, which is useful for converting a long-format DataFrame into a wide format.
Example
The following example demonstrates the working of the df.unstack() method for unstacking a DataFrame.
import pandas as pd import numpy as np # Create MultiIndex tuples = [["x", "x", "y", "y", "", "f", "z", "z"],["1", "2", "1", "2", "1", "2", "1", "2"]] index = pd.MultiIndex.from_arrays(tuples, names=["first", "second"]) # Create a DataFrame df = pd.DataFrame(np.random.randn(8, 2), index=index, columns=["A", "B"]) # Display the input DataFrame print('Input DataFrame:\n', df) # Unstack the DataFrame unstacked = df.unstack() print('Output Reshaped DataFrame:\n', unstacked)
Following is the output of the above code −
Input DataFrame:
A | B | ||
---|---|---|---|
first | second | ||
x | 1 | -0.407537 | -0.957010 |
2 | 0.045479 | 0.789849 | |
y | 1 | 0.751488 | -0.474536 |
2 | -1.043122 | -0.015152 | |
1 | -0.133349 | 1.094900 | |
f | 2 | 1.681111 | 2.480652 |
z | 1 | 0.283679 | 0.769553 |
2 | -2.034907 | 0.301275 |
Handling Missing Data during Unstacking
Unstacking can produce missing values when the reshaped DataFrame has unequal label sets in subgroups. Pandas handles these missing values with NaN by default, but you can specify a custom fill value.
Example
This example demonstrates how to handle missing values when unstacking a DataFrame.
import pandas as pd import numpy as np # Create Data index = pd.MultiIndex.from_product([["bar", "baz", "foo", "qux"], ["one", "two"]], names=["first", "second"]) columns = pd.MultiIndex.from_tuples([("A", "cat"), ("B", "dog"), ("B", "cat"), ("A", "dog")], names=["exp", "animal"]) df = pd.DataFrame(np.random.randn(8, 4), index=index, columns=columns) # Create a DataFrame df3 = df.iloc[[0, 1, 4, 7], [1, 2]] print(df3) # Unstack the DataFame unstacked = df3.unstack() # Display the Unstacked DataFrame print("Unstacked DataFrame without Filling:\n",unstacked) unstacked_filled = df3.unstack(fill_value=1) print("Unstacked DataFrame with Filling:\n",unstacked_filled)
Following is the output of the above code −
exp B animal dog cat first second bar one -0.556587 -0.157084 two 0.109060 0.856019 foo one -1.034260 1.548955 qux two -0.644370 -1.871248 Unstacked DataFrame without Filling: exp B animal dog cat second one two one two first bar -0.556587 0.10906 -0.157084 0.856019 foo -1.034260 NaN 1.548955 NaN qux NaN -0.64437 NaN -1.871248 Unstacked DataFrame with Filling: exp B animal dog cat second one two one two first bar -0.556587 0.10906 -0.157084 0.856019 foo -1.034260 1.00000 1.548955 1.000000 qux 1.000000 -0.64437 1.000000 -1.871248