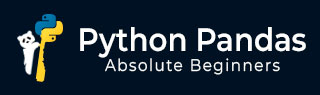
- Python Pandas - Home
- Python Pandas - Introduction
- Python Pandas - Environment Setup
- Python Pandas - Basics
- Python Pandas - Introduction to Data Structures
- Python Pandas - Index Objects
- Python Pandas - Panel
- Python Pandas - Basic Functionality
- Python Pandas - Indexing & Selecting Data
- Python Pandas - Series
- Python Pandas - Series
- Python Pandas - Slicing a Series Object
- Python Pandas - Attributes of a Series Object
- Python Pandas - Arithmetic Operations on Series Object
- Python Pandas - Converting Series to Other Objects
- Python Pandas - DataFrame
- Python Pandas - DataFrame
- Python Pandas - Accessing DataFrame
- Python Pandas - Slicing a DataFrame Object
- Python Pandas - Modifying DataFrame
- Python Pandas - Removing Rows from a DataFrame
- Python Pandas - Arithmetic Operations on DataFrame
- Python Pandas - IO Tools
- Python Pandas - IO Tools
- Python Pandas - Working with CSV Format
- Python Pandas - Reading & Writing JSON Files
- Python Pandas - Reading Data from an Excel File
- Python Pandas - Writing Data to Excel Files
- Python Pandas - Working with HTML Data
- Python Pandas - Clipboard
- Python Pandas - Working with HDF5 Format
- Python Pandas - Comparison with SQL
- Python Pandas - Data Handling
- Python Pandas - Sorting
- Python Pandas - Reindexing
- Python Pandas - Iteration
- Python Pandas - Concatenation
- Python Pandas - Statistical Functions
- Python Pandas - Descriptive Statistics
- Python Pandas - Working with Text Data
- Python Pandas - Function Application
- Python Pandas - Options & Customization
- Python Pandas - Window Functions
- Python Pandas - Aggregations
- Python Pandas - Merging/Joining
- Python Pandas - MultiIndex
- Python Pandas - Basics of MultiIndex
- Python Pandas - Indexing with MultiIndex
- Python Pandas - Advanced Reindexing with MultiIndex
- Python Pandas - Renaming MultiIndex Labels
- Python Pandas - Sorting a MultiIndex
- Python Pandas - Binary Operations
- Python Pandas - Binary Comparison Operations
- Python Pandas - Boolean Indexing
- Python Pandas - Boolean Masking
- Python Pandas - Data Reshaping & Pivoting
- Python Pandas - Pivoting
- Python Pandas - Stacking & Unstacking
- Python Pandas - Melting
- Python Pandas - Computing Dummy Variables
- Python Pandas - Categorical Data
- Python Pandas - Categorical Data
- Python Pandas - Ordering & Sorting Categorical Data
- Python Pandas - Comparing Categorical Data
- Python Pandas - Handling Missing Data
- Python Pandas - Missing Data
- Python Pandas - Filling Missing Data
- Python Pandas - Interpolation of Missing Values
- Python Pandas - Dropping Missing Data
- Python Pandas - Calculations with Missing Data
- Python Pandas - Handling Duplicates
- Python Pandas - Duplicated Data
- Python Pandas - Counting & Retrieving Unique Elements
- Python Pandas - Duplicated Labels
- Python Pandas - Grouping & Aggregation
- Python Pandas - GroupBy
- Python Pandas - Time-series Data
- Python Pandas - Date Functionality
- Python Pandas - Timedelta
- Python Pandas - Sparse Data Structures
- Python Pandas - Sparse Data
- Python Pandas - Visualization
- Python Pandas - Visualization
- Python Pandas - Additional Concepts
- Python Pandas - Caveats & Gotchas
Python Pandas - Dropping Missing Data
Missing data is a common issue when working with real-world datasets. The Python Pandas library provides an easy way for removing rows or columns that contain missing values (NaN or NaT) from a dataset using the dropna() method.
The dropna() method in Pandas is a useful tool to handle missing data by dropping rows or columns based on your specific requirements. In this tutorial, we will learn how to use dropna() to clean your dataset by dropping missing data based on various conditions.
The dropna() Method
The Pandas dropna() method allows you to remove missing values from a Pandas data structures such as, Series and DataFrame objects. It offers several options to customize how you drop rows or columns based on the presence of NaN values. This method returns a new Pandas object with missing data dropped or it returns None if inplace parameter is set to True.
Syntax
Following is the syntax −
DataFrame.dropna(*, axis=0, how=<no_default>, thresh=<no_default>, subset=None, inplace=False, ignore_index=False)
Where,
axis: 0 or 'index' (default) to drop rows; 1 or 'columns' to drop columns.
how: By default it is set to 'any', which drops that row or column if any missing values are present. If set to 'all', then it drops that row or column if all the missing values.
thresh: Require a minimum number of non-NA values to retain the row or column.
subset: List of specific columns (if dropping rows) or rows (if dropping columns) to consider.
inplace: Modify the DataFrame in place (default is False).
ignore_indexReset the index of the result (default is False).
Let's explore the how of the dropna() method drops the missing data based on various conditions.
Drop Rows with Any Missing Values
By default, the dropna() method removes rows where any missing values are present.
Example
The following example uses the dropna() method to drop the rows that have any missing values.
import pandas as pd import numpy as np dataset = {"Student_name": ["Ajay", "Krishna", "Deepak", "Swati"], "Roll_number": [23, 45, np.nan, 18], "Major_Subject": ["Maths", "Physics", "Arts", "Political science"], "Marks": [57, np.nan, 98, np.nan]} df = pd.DataFrame(dataset, index= [1, 2, 3, 4]) print("Original DataFrame:") print(df) # Drop the rows that have any missing values df_cleaned = df.dropna() print('\nResultant DataFrame after removing row:\n',df_cleaned)
Following is the output of the above code −
Original DataFrame:
Student name | Roll number | Major Subject | Marks | |
---|---|---|---|---|
1 | Ajay | 23.0 | Maths | 57.0 |
2 | Krishna | 45.0 | Physics | NaN |
3 | Deepak | NaN | Arts | 98.0 |
4 | Swati | 18.0 | Political science | NaN |
Student name | Roll number | Major Subject | Marks | |
---|---|---|---|---|
1 | Ajay | 23.0 | Maths | 57.0 |
Drop Rows Where All Values Are Missing
To drop rows where all values are missing, then we need to set how='all' parameter to the dropna() method.
Example
The following example demonstrates how to drop the rows where all values are missing in a DataFrame.
import pandas as pd import numpy as np dataset = {"Student name": ["Ajay", np.nan, "Deepak", "Swati"], "Roll number": [23, np.nan, np.nan, 18], "Major Subject": ["Maths", np.nan, "Arts", "Political science"], "Marks": [57, np.nan, 98, np.nan]} df = pd.DataFrame(dataset, index= [1, 2, 3, 4]) print("Original DataFrame:") print(df) # Drop rows where all values are missing reslut = df.dropna(how='all') print('\nResultant DataFrame after removing row:\n',reslut)
Following is the output of the above code −
Original DataFrame:
Student name | Roll number | Major Subject | Marks | |
---|---|---|---|---|
1 | Ajay | 23.0 | Maths | 57.0 |
2 | NaN | NaN | NaN | NaN |
3 | Deepak | NaN | Arts | 98.0 |
4 | Swati | 18.0 | Political science | NaN |
Student name | Roll number | Major Subject | Marks | |
---|---|---|---|---|
1 | Ajay | 23.0 | Maths | 57.0 |
3 | Deepak | NaN | Arts | 98.0 |
4 | Swati | 18.0 | Political science | NaN |
Keep Rows with a Minimum Number of Missing Values
The pandas dropan() method provides the thresh parameter to specify a minimum threshold of non-missing values for keeping rows with a minimum number of Non-Na values.
Example
This example demonstrates how to keep the rows the minimum number of missing values.
import pandas as pd import numpy as np dataset = {"Student name": ["Ajay", "Krishna", "Deepak", "Swati"], "Roll number": [23, np.nan, np.nan, 18], "Major Subject": ["Maths", np.nan, "Arts", "Political science"], "Marks": [57, np.nan, 98, np.nan]} df = pd.DataFrame(dataset, index= [1, 2, 3, 4]) print("Original DataFrame:") print(df) # Drop the rows with a threshold result = df.dropna(thresh=2) print('\nResultant DataFrame after removing row:\n',result)
Following is the output of the above code −
Original DataFrame:
Student name | Roll number | Major Subject | Marks | |
---|---|---|---|---|
1 | Ajay | 23.0 | Maths | 57.0 |
2 | Krishna | NaN | NaN | NaN |
3 | Deepak | NaN | Arts | 98.0 |
4 | Swati | 18.0 | Political science | NaN |
Student name | Roll number | Major Subject | Marks | |
---|---|---|---|---|
1 | Ajay | 23.0 | Maths | 57.0 |
3 | Deepak | NaN | Arts | 98.0 |
4 | Swati | 18.0 | Political science | NaN |
Drop Columns with Any Missing Values
To drop columns that contain any missing values, then we can use axis parameter of the dropna() method to select the columns.
Example
This example show how the dropna() method removes the entire column where any of the value is missing.
import pandas as pd import numpy as np dataset = {"Student_name": ["Ajay", "Krishna", "Deepak", "Swati"], "Roll_number": [23, 45, np.nan, 18], "Major_Subject": ["Maths", "Physics", "Arts", "Political science"], "Marks": [57, np.nan, 98, np.nan]} df = pd.DataFrame(dataset, index= [1, 2, 3, 4]) print("Original DataFrame:") print(df) # Drop column with any missing values result = df.dropna(axis='columns') print('\nResultant DataFrame after removing columns:\n',result)
Following is the output of the above code −
Original DataFrame:
Student name | Roll number | Major Subject | Marks | |
---|---|---|---|---|
1 | Ajay | 23.0 | Maths | 57.0 |
2 | Krishna | 45.0 | Physics | NaN |
3 | Deepak | NaN | Arts | 98.0 |
4 | Swati | 18.0 | Political science | NaN |
Student name | Major Subject | |
---|---|---|
1 | Ajay | Maths |
2 | Krishna | Physics |
3 | Deepak | Arts |
4 | Swati | Political science |
Drop Rows Based on Missing Data in Specific Columns
You can use the subset parameter of the drop() method to focus only on those particular columns while dropping rows where data is missing.
Example
This example shows how to remove the rows based on missing data present in the specific column using the subset parameter of the dropna() method.
import pandas as pd import numpy as np dataset = {"Student_name": ["Ajay", "Krishna", "Deepak", "Swati"], "Roll_number": [23, 45, np.nan, 18], "Major_Subject": ["Maths", "Physics", np.nan, "Political science"], "Marks": [57, np.nan, 98, np.nan]} df = pd.DataFrame(dataset, index= [1, 2, 3, 4]) print("Original DataFrame:") print(df) # Drop Rows Based on Missing Data in Specific Columns result = df.dropna(subset=['Roll_number', 'Major_Subject']) print('\nResultant DataFrame after removing rows:\n',result)
Following is the output of the above code −
Original DataFrame:
Student name | Roll number | Major Subject | Marks | |
---|---|---|---|---|
1 | Ajay | 23.0 | Maths | 57.0 |
2 | Krishna | 45.0 | Physics | NaN |
3 | Deepak | NaN | NaN | 98.0 |
4 | Swati | 18.0 | Political science | NaN |
Student name | Roll number | Major Subject | Marks | |
---|---|---|---|---|
1 | Ajay | 23.0 | Maths | 57.0 |
2 | Krishna | 45.0 | Physics | NaN |
4 | Swati | 18.0 | Political science | NaN |