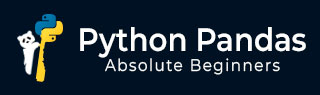
- Python Pandas - Home
- Python Pandas - Introduction
- Python Pandas - Environment Setup
- Python Pandas - Basics
- Python Pandas - Introduction to Data Structures
- Python Pandas - Index Objects
- Python Pandas - Panel
- Python Pandas - Basic Functionality
- Python Pandas - Indexing & Selecting Data
- Python Pandas - Series
- Python Pandas - Series
- Python Pandas - Slicing a Series Object
- Python Pandas - Attributes of a Series Object
- Python Pandas - Arithmetic Operations on Series Object
- Python Pandas - Converting Series to Other Objects
- Python Pandas - DataFrame
- Python Pandas - DataFrame
- Python Pandas - Accessing DataFrame
- Python Pandas - Slicing a DataFrame Object
- Python Pandas - Modifying DataFrame
- Python Pandas - Removing Rows from a DataFrame
- Python Pandas - Arithmetic Operations on DataFrame
- Python Pandas - IO Tools
- Python Pandas - IO Tools
- Python Pandas - Working with CSV Format
- Python Pandas - Reading & Writing JSON Files
- Python Pandas - Reading Data from an Excel File
- Python Pandas - Writing Data to Excel Files
- Python Pandas - Working with HTML Data
- Python Pandas - Clipboard
- Python Pandas - Working with HDF5 Format
- Python Pandas - Comparison with SQL
- Python Pandas - Data Handling
- Python Pandas - Sorting
- Python Pandas - Reindexing
- Python Pandas - Iteration
- Python Pandas - Concatenation
- Python Pandas - Statistical Functions
- Python Pandas - Descriptive Statistics
- Python Pandas - Working with Text Data
- Python Pandas - Function Application
- Python Pandas - Options & Customization
- Python Pandas - Window Functions
- Python Pandas - Aggregations
- Python Pandas - Merging/Joining
- Python Pandas - MultiIndex
- Python Pandas - Basics of MultiIndex
- Python Pandas - Indexing with MultiIndex
- Python Pandas - Advanced Reindexing with MultiIndex
- Python Pandas - Renaming MultiIndex Labels
- Python Pandas - Sorting a MultiIndex
- Python Pandas - Binary Operations
- Python Pandas - Binary Comparison Operations
- Python Pandas - Boolean Indexing
- Python Pandas - Boolean Masking
- Python Pandas - Data Reshaping & Pivoting
- Python Pandas - Pivoting
- Python Pandas - Stacking & Unstacking
- Python Pandas - Melting
- Python Pandas - Computing Dummy Variables
- Python Pandas - Categorical Data
- Python Pandas - Categorical Data
- Python Pandas - Ordering & Sorting Categorical Data
- Python Pandas - Comparing Categorical Data
- Python Pandas - Handling Missing Data
- Python Pandas - Missing Data
- Python Pandas - Filling Missing Data
- Python Pandas - Interpolation of Missing Values
- Python Pandas - Dropping Missing Data
- Python Pandas - Calculations with Missing Data
- Python Pandas - Handling Duplicates
- Python Pandas - Duplicated Data
- Python Pandas - Counting & Retrieving Unique Elements
- Python Pandas - Duplicated Labels
- Python Pandas - Grouping & Aggregation
- Python Pandas - GroupBy
- Python Pandas - Time-series Data
- Python Pandas - Date Functionality
- Python Pandas - Timedelta
- Python Pandas - Sparse Data Structures
- Python Pandas - Sparse Data
- Python Pandas - Visualization
- Python Pandas - Visualization
- Python Pandas - Additional Concepts
- Python Pandas - Caveats & Gotchas
Python Pandas - Filling Missing Data
Filling missing data is a process of replacing the missing (NaN) values with meaningful alternatives. Whether you want to replace missing values with a constant value, or propagate the values forward or backward, Pandas has built-in functions to achieve this.
In this tutorial, we'll learn different ways to fill missing data in Pandas including −
Replacing missing values with a scalar.
Forward and backward filling.
Using a specified limit for filling.
Replacing Data with the replace() method.
Replacing values with regular expressions.
Filling Missing Data with Scalar Value
The fillna() method in Pandas is used to fill missing values (NA or NaN) with a scalar value, such as any specific number.
Example
The following demonstrates how to fill the missing values NaN with a scalar value ("NaN" with "5") using the fillna() method.
import pandas as pd import numpy as np # Create DataFrame with missing values data = {"Col1": [3, np.nan, np.nan, 2], "Col2": [1.0, pd.NA, pd.NA, 2.0]} df = pd.DataFrame(data) # Display the original DataFrame with missing values print("Original DataFrame:\n",df) # Fill missing values with 5 df_filled = df.fillna(5) print("\nResultant DataFrame after NaN replaced with '5':\n", df_filled)
Its output is as follows −
Original DataFrame:
Col1 | Col2 | |
---|---|---|
0 | 3.0 | 1.0 |
1 | NaN | <NA> |
2 | NaN | <NA> |
3 | 2.0 | 2.0 |
Col1 | Col2 | |
---|---|---|
0 | 3.0 | 1.0 |
1 | 5.0 | 5.0 |
2 | 5.0 | 5.0 |
3 | 2.0 | 2.0 |
Filling Missing Values Forward or Backward
You can also propagate the last valid observation forward or backward to fill gaps using the ffill() and bfill() methods respectively.
Sr.No | Method & Action |
---|---|
1 | ffill() This method fills missing values with the previous valid value. |
2 | bfill() This methods fills missing values with the next valid value. |
Example: Forward Fill
This example replaces the missing values with the forward fill ffill() method.
import pandas as pd import numpy as np # Create DataFrame with missing values df = pd.DataFrame([[9, -3, -2], [-5, 1, 8], [6, 4, -8]], index=['a', 'c', 'd'], columns=['one', 'two', 'three']) df = df.reindex(['a', 'b', 'c', 'd', 'e']) # Display the original DataFrame with missing values print("Original DataFrame:\n",df) # Forward Fill the missing values result = df.ffill() print("\nResultant DataFrame after Forward fill:\n", result)
Its output is as follows −
Original DataFrame:
one | two | three | |
---|---|---|---|
a | 9.0 | -3.0 | -2.0 |
b | NaN | NaN | NaN |
c | -5.0 | 1.0 | 8.0 |
d | 6.0 | 4.0 | -8.0 |
e | NaN | NaN | NaN |
one | two | three | |
---|---|---|---|
a | 9.0 | -3.0 | -2.0 |
b | 9.0 | -3.0 | -2.0 |
c | -5.0 | 1.0 | 8.0 |
d | 6.0 | 4.0 | -8.0 |
e | 6.0 | 4.0 | -8.0 |
Example: Backward Fill
This example replaces the missing values with backward fill bfill() method.
import pandas as pd import numpy as np # Create DataFrame with missing values df = pd.DataFrame([[9, -3, -2], [-5, 1, 8], [6, 4, -8]], index=['a', 'c', 'd'], columns=['one', 'two', 'three']) df = df.reindex(['a', 'b', 'c', 'd', 'e']) # Display the original DataFrame with missing values print("Original DataFrame:\n",df) # Backward Fill the missing values result = df.bfill() print("\nResultant DataFrame after Backward fill:\n", result)
Its output is as follows −
Original DataFrame:
one | two | three | |
---|---|---|---|
a | 9.0 | -3.0 | -2.0 |
b | NaN | NaN | NaN |
c | -5.0 | 1.0 | 8.0 |
d | 6.0 | 4.0 | -8.0 |
e | NaN | NaN | NaN |
one | two | three | |
---|---|---|---|
a | 9.0 | -3.0 | -2.0 |
b | -5.0 | 1.0 | 8.0 |
c | -5.0 | 1.0 | 8.0 |
d | 6.0 | 4.0 | -8.0 |
e | NaN | NaN | NaN |
Limiting the Number of Fills
You can also control the limit of how many consecutive missing values are filled by specifying the limit parameter.
Example
The following example demonstrates how to set limit for filling the missing values using the ffill() method with the limit parameter.
import pandas as pd import numpy as np # Create DataFrame with missing values df = pd.DataFrame([[9, -3, -2], [-5, 1, 8], [6, 4, -8]], index=['a', 'c', 'd'], columns=['one', 'two', 'three']) df = df.reindex(['a', 'b', 'd', 'e', 'f']) # Display the original DataFrame with missing values print("Original DataFrame:\n",df) # Forward Fill the missing values with limit result = df.ffill(limit=1) print("\nResultant DataFrame after Forward fill:\n", result)
Following is the output of the above code −
Original DataFrame:
one | two | three | |
---|---|---|---|
a | 9.0 | -3.0 | -2.0 |
b | NaN | NaN | NaN |
d | 6.0 | 4.0 | -8.0 |
e | NaN | NaN | NaN |
f | NaN | NaN | NaN |
one | two | three | |
---|---|---|---|
a | 9.0 | -3.0 | -2.0 |
b | 9.0 | -3.0 | -2.0 |
d | 6.0 | 4.0 | -8.0 |
e | 6.0 | 4.0 | -8.0 |
f | NaN | NaN | NaN |
Replacing Data with the replace() method
Many times, we have to replace a generic value with some specific value. We can achieve this by applying the replace() method.
Replacing NA with a scalar value is equivalent behavior of the fillna() function.
Example
Here is the example of replacing the generic values using the replace() method.
import pandas as pd import numpy as np # Create DataFrame df = pd.DataFrame({'one':[10,20,30,40,50,2000], 'two':[1000,0,30,40,50,60]}) # Replace the generic values print(df.replace({1000:10,2000:60}))
Its output is as follows −
one | two | |
---|---|---|
0 | 10 | 10 |
1 | 20 | 0 |
2 | 30 | 30 |
3 | 40 | 40 |
4 | 50 | 50 |
5 | 60 | 60 |
Replacing Missing Data Using Regular Expressions
You can also use regex patterns to replace the missing values in your data with the replace() method.
Example
Here is the example of replacing the a specific data using the regular expression with the replace() method.
import pandas as pd import numpy as np # Create DataFrame with missing values df = pd.DataFrame({"a": list(range(4)), "b": list("ab.."), "c": ["a", "b", np.nan, "d"]}) # Display the original DataFrame with missing values print("Original DataFrame:\n",df) # Replace the missing values with regular exp result = df.replace(r"\.", 10, regex=True) print("\nResultant DataFrame after filling the missing values using regex:\n", result)
Its output is as follows −
Original DataFrame:
a | b | c | |
---|---|---|---|
0 | 0 | a | a |
1 | 1 | b | b |
2 | 2 | . | NaN |
3 | 3 | . | d |
a | b | c | |
---|---|---|---|
0 | 0 | a | a |
1 | 1 | b | b |
2 | 2 | 10 | NaN |
3 | 3 | 10 | d |