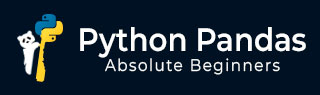
- Python Pandas - Home
- Python Pandas - Introduction
- Python Pandas - Environment Setup
- Python Pandas - Basics
- Python Pandas - Introduction to Data Structures
- Python Pandas - Index Objects
- Python Pandas - Panel
- Python Pandas - Basic Functionality
- Python Pandas - Indexing & Selecting Data
- Python Pandas - Series
- Python Pandas - Series
- Python Pandas - Slicing a Series Object
- Python Pandas - Attributes of a Series Object
- Python Pandas - Arithmetic Operations on Series Object
- Python Pandas - Converting Series to Other Objects
- Python Pandas - DataFrame
- Python Pandas - DataFrame
- Python Pandas - Accessing DataFrame
- Python Pandas - Slicing a DataFrame Object
- Python Pandas - Modifying DataFrame
- Python Pandas - Removing Rows from a DataFrame
- Python Pandas - Arithmetic Operations on DataFrame
- Python Pandas - IO Tools
- Python Pandas - IO Tools
- Python Pandas - Working with CSV Format
- Python Pandas - Reading & Writing JSON Files
- Python Pandas - Reading Data from an Excel File
- Python Pandas - Writing Data to Excel Files
- Python Pandas - Working with HTML Data
- Python Pandas - Clipboard
- Python Pandas - Working with HDF5 Format
- Python Pandas - Comparison with SQL
- Python Pandas - Data Handling
- Python Pandas - Sorting
- Python Pandas - Reindexing
- Python Pandas - Iteration
- Python Pandas - Concatenation
- Python Pandas - Statistical Functions
- Python Pandas - Descriptive Statistics
- Python Pandas - Working with Text Data
- Python Pandas - Function Application
- Python Pandas - Options & Customization
- Python Pandas - Window Functions
- Python Pandas - Aggregations
- Python Pandas - Merging/Joining
- Python Pandas - MultiIndex
- Python Pandas - Basics of MultiIndex
- Python Pandas - Indexing with MultiIndex
- Python Pandas - Advanced Reindexing with MultiIndex
- Python Pandas - Renaming MultiIndex Labels
- Python Pandas - Sorting a MultiIndex
- Python Pandas - Binary Operations
- Python Pandas - Binary Comparison Operations
- Python Pandas - Boolean Indexing
- Python Pandas - Boolean Masking
- Python Pandas - Data Reshaping & Pivoting
- Python Pandas - Pivoting
- Python Pandas - Stacking & Unstacking
- Python Pandas - Melting
- Python Pandas - Computing Dummy Variables
- Python Pandas - Categorical Data
- Python Pandas - Categorical Data
- Python Pandas - Ordering & Sorting Categorical Data
- Python Pandas - Comparing Categorical Data
- Python Pandas - Handling Missing Data
- Python Pandas - Missing Data
- Python Pandas - Filling Missing Data
- Python Pandas - Interpolation of Missing Values
- Python Pandas - Dropping Missing Data
- Python Pandas - Calculations with Missing Data
- Python Pandas - Handling Duplicates
- Python Pandas - Duplicated Data
- Python Pandas - Counting & Retrieving Unique Elements
- Python Pandas - Duplicated Labels
- Python Pandas - Grouping & Aggregation
- Python Pandas - GroupBy
- Python Pandas - Time-series Data
- Python Pandas - Date Functionality
- Python Pandas - Timedelta
- Python Pandas - Sparse Data Structures
- Python Pandas - Sparse Data
- Python Pandas - Visualization
- Python Pandas - Visualization
- Python Pandas - Additional Concepts
- Python Pandas - Caveats & Gotchas
Python Pandas - Categorical Data
In pandas, categorical data refers to a data type that represents categorical variables, similar to the concept of factors in R. It is a specialized data type designed for handling categorical variables, commonly used in statistics. A categorical variable can represent values like "male" or "female," or ratings on a scale such as "poor," "average," and "excellent." Unlike numerical data, you cannot perform mathematical operations like addition or division on categorical data.
In Pandas, categorical data is stored more efficiently because it uses a combination of an array of category values and an array of integer codes that refer to those categories. This saves memory and improves performance when working with large datasets containing repeated values.
The categorical data type is useful in the following cases −
A string variable consisting of only a few different values. Converting such a string variable to a categorical variable will save some memory.
The lexical order of a variable is not the same as the logical order (one, two, three). By converting to a categorical and specifying an order on the categories, sorting and min/max will use the logical order instead of the lexical order.
As a signal to other python libraries that this column should be treated as a categorical variable (e.g. to use suitable statistical methods or plot types).
In this tutorial we will learn about basics of working with categorical data in Pandas, including series and DataFrame creation, controlling behavior, and regaining original data from categorical values.
Series and DataFrame Creation with Categorical Data
Pandas Series or DataFrame object can be created directly with the categorical data using the dtype="category" parameter of the Pandas Series() or DataFrame() constructors.
Example: Series Creation with Categorical Data
Following is the basic example of creating a Pandas Series object with the categorical data.
import pandas as pd # Create Series object with categorical data s = pd.Series(["a", "b", "c", "a"], dtype="category") # Display the categorical Series print('Series with Categorical Data:\n', s)
Following is the output of the above code −
Series with Categorical Data: 0 a 1 b 2 c 3 a dtype: category Categories (3, object): ['a', 'b', 'c']
Example: Converting an Existing DataFrame Column to Categorical
This example demonstrates converting an existing Pandas DataFrame column to categorical data type using the astype() method.
import pandas as pd import numpy as np # Create a DataFrame df = pd.DataFrame({"Col_a": list("aeeioou"), "Col_b": range(7)}) # Display the Input DataFrame print('Input DataFrame:\n',df) print('\nVerify the Data type of each column:\n', df.dtypes) # Convert the Data type of col_a to categorical df['Col_a'] = df["Col_a"].astype("category") # Display the Input DataFrame print('\nConverted DataFrame:\n',df) print('\nVerify the Data type of each column:\n', df.dtypes)
Following is the output of the above code −
Input DataFrame:Col_a | Col_b | |
---|---|---|
0 | a | 0 |
1 | e | 1 |
2 | e | 2 |
3 | i | 3 |
4 | o | 4 |
5 | o | 5 |
6 | u | 6 |
Verify the Data type of each column: Col_a object Col_b int64 dtype: objectConverted DataFrame:
Col_a | Col_b | |
---|---|---|
0 | a | 0 |
1 | e | 1 |
2 | e | 2 |
3 | i | 3 |
4 | o | 4 |
5 | o | 5 |
6 | u | 6 |
Verify the Data type of each column: Col_a category Col_b int64 dtype: object
Controlling Behavior of the Categorical Data
By default, Pandas infers categories from the data and treats them as unordered. To control the behavior, you can use the CategoricalDtype class from the pandas.api.types module.
Example
This example demonstrates how to apply the CategoricalDtype to a whole DataFrame.
import pandas as pd from pandas.api.types import CategoricalDtype # Create a DataFrame df = pd.DataFrame({"A": list("abca"), "B": list("bccd")}) # Display the Input DataFrame print('Input DataFrame:\n',df) print('\nVerify the Data type of each column:\n', df.dtypes) # Applying CategoricalDtype to a DataFrame cat_type = CategoricalDtype(categories=list("abcd"), ordered=True) df_cat = df.astype(cat_type) # Display the Input DataFrame print('\nConverted DataFrame:\n', df_cat) print('\nVerify the Data type of each column:\n', df_cat.dtypes)
Following is the output of the above code −
Input DataFrame:A | B | |
---|---|---|
0 | a | b |
1 | b | c |
2 | c | c |
3 | a | d |
Verify the Data type of each column: A object B object dtype: objectConverted DataFrame:
A | B | |
---|---|---|
0 | a | b |
1 | b | c |
2 | c | c |
3 | a | d |
Verify the Data type of each column: A category B category
Converting the Categorical Data Back to Original
After converting a Series to categorical data, you can convert it back to its original form using Series.astype() or np.asarray().
Example
This example converts the categorical data of Series object back to the object data type using the astype() method.
import pandas as pd # Create Series object with categorical data s = pd.Series(["a", "b", "c", "a"], dtype="category") # Display the categorical Series print('Series with Categorical Data:\n', s) # Display the converted Series print('Converted Series back to original:\n ', s.astype(str))
Following is the output of the above code −
Series with Categorical Data: 0 a 1 b 2 c 3 a dtype: category Categories (3, object): ['a', 'b', 'c'] Converted Series back to original: 0 a 1 b 2 c 3 a dtype: object
Description to a Data Column
Using the .describe() command on the categorical data, we get similar output to a Series or DataFrame of the type string.
Example
The following example demonstrates how to get the description of Pandas categorical DataFrame using the describe() method.
import pandas as pd import numpy as np cat = pd.Categorical(["a", "c", "c", np.nan], categories=["b", "a", "c"]) df = pd.DataFrame({"cat":cat, "s":["a", "c", "c", np.nan]}) print("Description for whole DataFrame:") print(df.describe()) print("\nDescription only for a DataFrame column:") print(df["cat"].describe())
Its output is as follows −
Description for whole DataFrame:cat | s | ||
---|---|---|---|
count | 3 | 3 | |
unique | 2 | 2 | |
top | c | c | |
freq | 2 | 2 |
Description only for a DataFrame column: count 3 unique 2 top c freq 2 Name: cat, dtype: object