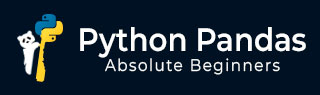
- Python Pandas - Home
- Python Pandas - Introduction
- Python Pandas - Environment Setup
- Python Pandas - Basics
- Python Pandas - Introduction to Data Structures
- Python Pandas - Index Objects
- Python Pandas - Panel
- Python Pandas - Basic Functionality
- Python Pandas - Indexing & Selecting Data
- Python Pandas - Series
- Python Pandas - Series
- Python Pandas - Slicing a Series Object
- Python Pandas - Attributes of a Series Object
- Python Pandas - Arithmetic Operations on Series Object
- Python Pandas - Converting Series to Other Objects
- Python Pandas - DataFrame
- Python Pandas - DataFrame
- Python Pandas - Accessing DataFrame
- Python Pandas - Slicing a DataFrame Object
- Python Pandas - Modifying DataFrame
- Python Pandas - Removing Rows from a DataFrame
- Python Pandas - Arithmetic Operations on DataFrame
- Python Pandas - IO Tools
- Python Pandas - IO Tools
- Python Pandas - Working with CSV Format
- Python Pandas - Reading & Writing JSON Files
- Python Pandas - Reading Data from an Excel File
- Python Pandas - Writing Data to Excel Files
- Python Pandas - Working with HTML Data
- Python Pandas - Clipboard
- Python Pandas - Working with HDF5 Format
- Python Pandas - Comparison with SQL
- Python Pandas - Data Handling
- Python Pandas - Sorting
- Python Pandas - Reindexing
- Python Pandas - Iteration
- Python Pandas - Concatenation
- Python Pandas - Statistical Functions
- Python Pandas - Descriptive Statistics
- Python Pandas - Working with Text Data
- Python Pandas - Function Application
- Python Pandas - Options & Customization
- Python Pandas - Window Functions
- Python Pandas - Aggregations
- Python Pandas - Merging/Joining
- Python Pandas - MultiIndex
- Python Pandas - Basics of MultiIndex
- Python Pandas - Indexing with MultiIndex
- Python Pandas - Advanced Reindexing with MultiIndex
- Python Pandas - Renaming MultiIndex Labels
- Python Pandas - Sorting a MultiIndex
- Python Pandas - Binary Operations
- Python Pandas - Binary Comparison Operations
- Python Pandas - Boolean Indexing
- Python Pandas - Boolean Masking
- Python Pandas - Data Reshaping & Pivoting
- Python Pandas - Pivoting
- Python Pandas - Stacking & Unstacking
- Python Pandas - Melting
- Python Pandas - Computing Dummy Variables
- Python Pandas - Categorical Data
- Python Pandas - Categorical Data
- Python Pandas - Ordering & Sorting Categorical Data
- Python Pandas - Comparing Categorical Data
- Python Pandas - Handling Missing Data
- Python Pandas - Missing Data
- Python Pandas - Filling Missing Data
- Python Pandas - Interpolation of Missing Values
- Python Pandas - Dropping Missing Data
- Python Pandas - Calculations with Missing Data
- Python Pandas - Handling Duplicates
- Python Pandas - Duplicated Data
- Python Pandas - Counting & Retrieving Unique Elements
- Python Pandas - Duplicated Labels
- Python Pandas - Grouping & Aggregation
- Python Pandas - GroupBy
- Python Pandas - Time-series Data
- Python Pandas - Date Functionality
- Python Pandas - Timedelta
- Python Pandas - Sparse Data Structures
- Python Pandas - Sparse Data
- Python Pandas - Visualization
- Python Pandas - Visualization
- Python Pandas - Additional Concepts
- Python Pandas - Caveats & Gotchas
Python Pandas - Read JSON
JavaScript Object Notation (JSON) is a popular data-interchange format for exchanging structured data. Python's Pandas library provides an easy-to-use read_json() method for reading JSON data into its powerful DataFrame or Series objects.
In this tutorial, we see advanced options for reading JSON data into Pandas objects, which includes working with nested JSON structures, handling line-delimited JSON, managing data types, and parsing dates using the pandas.read_json() method efficiently and effectively.
Introduction to read_json() Method
The pandas.read_json() method reads JSON data from various sources (like local file, URL, or JSON string) and converts it into a Pandas object.
Syntax
The syntax of this method is as follows −
pandas.read_json(path_or_buf, *, orient=None, typ='frame', dtype=None, lines=False)
Below are the key Parameters of the method −
path_or_buf: The input JSON string, file path, URL, or file-like object.
typ: Specifies the type of Pandas object to return ("frame" for DataFrame or "series" for Series).
orient: Determines the expected format of the JSON string.
dtype: Defines the data type of columns.
lines: Indicates whether the file should be read as one JSON object per line.
Example
Let's see an example of reading a JSON data from a .json file.
import pandas as pd # Reading a JSON file df = pd.read_json('json_file.json') print("DataFrame from JSON File:") print(df)
When we run above program, it produces following result −
DataFrame from JSON File:
Car | Date_of_purchase | |
---|---|---|
0 | BMW | 2025-01-01 |
1 | Lexus | 2025-01-02 |
2 | Audi | 2025-01-07 |
3 | Mercedes | 2025-01-06 |
4 | Jaguar | 2025-01-09 |
5 | Bentley | 2025-01-22 |
Pandas Reading JSON With Different Orient Options
The orient parameter of the pandas.read_json() method controls the expected JSON string format, which effects how Pandas reads the data. Below are the supported formats −
split: Dict with keys index, columns, and data.
records: Reads the JSON data into list of dictionaries.
index: Dict of dicts with keys as row indices.
columns: Dict of dicts with keys as columns labels.
table: Adhering to the JSON Table Schema
Example
The following example demonstrates specifying the 'index' orient option for reading the JSON data into a Pandas DataFrame object using the pandas.read_json() method.
import pandas as pd from io import StringIO # Input JSON data json_data = '{"col1": {"row1": "a", "row2": "b"}, "col2": {"row1": "c", "row2": "d"}}' # JSON with 'index' orientation df = pd.read_json(StringIO(json_data), orient='index') print("Index Orient:") print(df)
Following is an output of the above code −
Index Orient:
row1 | row2 | |
---|---|---|
col1 | a | b |
col2 | c | d |
Pandas Reading Line-Delimited JSON
Line-delimited JSON contains multiple JSON objects separated by newline. In other words, each line of the line-delimited JSON file contains a piece of JSON Data. It is commonly used in streaming APIs and log data. Pandas supports this format with the lines=True argument of the pandas.read_json() method.
Example
This example demonstrates reading line-delimited JSON file using the lines=True parameter of the pandas.read_json() method.
import pandas as pd from io import StringIO # Input JSON data json_data = """ {"Col1": "a", "Col2": "b"} {"Col1": "c", "Col2": "d"} """ # Reading Line-Delimited JSON df = pd.read_json(StringIO(json_data), lines=True) print("DataFrame from Line-Delimited JSON:") print(df)
Following is an output of the above code −
DataFrame from Line-Delimited JSON:
Col1 | Col2 | |
---|---|---|
0 | a | b |
1 | c | d |
Handling Data Types
While importing JSON, Pandas attempts to infer data types automatically. However, some fields, like dates, may require explicit handling to ensure correct parsing. The dtype parameters provide fine control over specifying the data types for each field.
Example
The following example demonstrates reading the JSON data with specifying the data type using the dtype parameter of the read_json() method.
import pandas as pd from io import StringIO # Reading JSON with specified dtypes json_data = '[{"A": 1.5, "B": "text", "C": true, "D": "2025-01-01"}]' # Reading JSON with specified dtypes df = pd.read_json(StringIO(json_data), dtype={"A": "float32", "B": "string"}) # Display the output DataFrame from specified data types print("Data type of the output DataFrame") print(df.dtypes)
Following is an output of the above code −
Data type of the output DataFrame A float32 B string[python] C bool D object dtype: object
Reading Nested JSON Structures with Pandas
Nested JSON structures occur when JSON objects have arrays or dictionaries as values. Flattening these structures into a tabular format can be possible by using the Pandas json_normalize() method.
Example
In this example we will read the nested JSON structure into Pandas DataFrame using the json_normalize() method.
from pandas import json_normalize # Sample Nested JSON Structures nested_json = [ { "id": 1, "name": "kiran", "details": {"age": 30, "city": "Hyderabad"} }, {"id": 2, "name": "Priya", "details": {"age": 25, "city": "Mumbai"} }] # Reading Nested JSON into DataFrame df = json_normalize(nested_json) print("DataFrame from Nested JSON:") print(df)
While executing the above code we get the following output −
DataFrame from Nested JSON:
id | name | details.age | details.city | |
---|---|---|---|---|
0 | 1 | Kiran | 30 | Hyderabad |
1 | 2 | Priya | 25 | Mumbai |