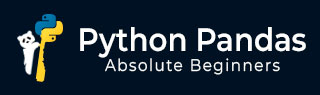
- Python Pandas - Home
- Python Pandas - Introduction
- Python Pandas - Environment Setup
- Python Pandas - Basics
- Python Pandas - Introduction to Data Structures
- Python Pandas - Index Objects
- Python Pandas - Panel
- Python Pandas - Basic Functionality
- Python Pandas - Indexing & Selecting Data
- Python Pandas - Series
- Python Pandas - Series
- Python Pandas - Slicing a Series Object
- Python Pandas - Attributes of a Series Object
- Python Pandas - Arithmetic Operations on Series Object
- Python Pandas - Converting Series to Other Objects
- Python Pandas - DataFrame
- Python Pandas - DataFrame
- Python Pandas - Accessing DataFrame
- Python Pandas - Slicing a DataFrame Object
- Python Pandas - Modifying DataFrame
- Python Pandas - Removing Rows from a DataFrame
- Python Pandas - Arithmetic Operations on DataFrame
- Python Pandas - IO Tools
- Python Pandas - IO Tools
- Python Pandas - Working with CSV Format
- Python Pandas - Reading & Writing JSON Files
- Python Pandas - Reading Data from an Excel File
- Python Pandas - Writing Data to Excel Files
- Python Pandas - Working with HTML Data
- Python Pandas - Clipboard
- Python Pandas - Working with HDF5 Format
- Python Pandas - Comparison with SQL
- Python Pandas - Data Handling
- Python Pandas - Sorting
- Python Pandas - Reindexing
- Python Pandas - Iteration
- Python Pandas - Concatenation
- Python Pandas - Statistical Functions
- Python Pandas - Descriptive Statistics
- Python Pandas - Working with Text Data
- Python Pandas - Function Application
- Python Pandas - Options & Customization
- Python Pandas - Window Functions
- Python Pandas - Aggregations
- Python Pandas - Merging/Joining
- Python Pandas - MultiIndex
- Python Pandas - Basics of MultiIndex
- Python Pandas - Indexing with MultiIndex
- Python Pandas - Advanced Reindexing with MultiIndex
- Python Pandas - Renaming MultiIndex Labels
- Python Pandas - Sorting a MultiIndex
- Python Pandas - Binary Operations
- Python Pandas - Binary Comparison Operations
- Python Pandas - Boolean Indexing
- Python Pandas - Boolean Masking
- Python Pandas - Data Reshaping & Pivoting
- Python Pandas - Pivoting
- Python Pandas - Stacking & Unstacking
- Python Pandas - Melting
- Python Pandas - Computing Dummy Variables
- Python Pandas - Categorical Data
- Python Pandas - Categorical Data
- Python Pandas - Ordering & Sorting Categorical Data
- Python Pandas - Comparing Categorical Data
- Python Pandas - Handling Missing Data
- Python Pandas - Missing Data
- Python Pandas - Filling Missing Data
- Python Pandas - Interpolation of Missing Values
- Python Pandas - Dropping Missing Data
- Python Pandas - Calculations with Missing Data
- Python Pandas - Handling Duplicates
- Python Pandas - Duplicated Data
- Python Pandas - Counting & Retrieving Unique Elements
- Python Pandas - Duplicated Labels
- Python Pandas - Grouping & Aggregation
- Python Pandas - GroupBy
- Python Pandas - Time-series Data
- Python Pandas - Date Functionality
- Python Pandas - Timedelta
- Python Pandas - Sparse Data Structures
- Python Pandas - Sparse Data
- Python Pandas - Visualization
- Python Pandas - Visualization
- Python Pandas - Additional Concepts
- Python Pandas - Caveats & Gotchas
Python Pandas read_html() Method
The Python Pandas read_html() method is a powerful tool to read tables from HTML documents and load them into a list of DataFrames. It supports multiple parsing engines (like lxml, BeautifulSoup) and provides extensive customization options through parameters like match, attrs, and extract_links. This method is particularly useful for web scraping and data analysis tasks that involve HTML tables.
HTML is a structured format used to represent tabular data in rows and columns within a webpage. Extracting tabular data from an HTML to Python's environment is possible by using this method.
Syntax
Below is the syntax of the read_html() method −
pandas.read_html(io, *, match='.+', flavor=None, header=None, index_col=None, skiprows=None, attrs=None, parse_dates=False, thousands=', ', encoding=None, decimal='.', converters=None, na_values=None, keep_default_na=True, displayed_only=True, extract_links=None, dtype_backend=<no_default>, storage_options=None)
Parameters
The Python Pandas read_html() method accepts following parameters −
io: A string, path object, or file-like object representing the HTML source or a URL.
match: A string or regex to filter tables based on matching text. Default is '.+'.
flavor: The parsing engine, e.g., 'lxml', 'html5lib', or 'bs4'.
header: Specifies row to use as column headers.
index_col: Column or list of columns to use as the DataFrame index.
skiprows: Rows to skip when parsing the table.
attrs: A dictionary of HTML table attributes for table selection.
parse_dates: Converts columns to datetime if set to True.
thousands: Specifies a separator to use to parse thousands. Defaults to ','.
encoding: Encoding used to decode the web page. By default it is set to None, which preserves the previous encoding.
decimal: Character to recognize as a decimal point.
converters: Functions to transform specific column values.
na_values: Customize NA values. Defaults to None.
extract_links: Extracts href links from table sections.
dtype_backend: Backend data type for the resultant DataFrame.
storage_options: Extra options related to storage connections.
Return Value
The Pandas read_html() method returns a list of DataFrames, where each DataFrame represents a table found in the HTML source.
Example: Reading an HTML String
The following example demonstrates the basic usage of the read_html() method to extract data from an HTML string.
import pandas as pd from io import StringIO # Create a string representing HTML table html_content = """ <table> <tr><th>Name</th><th>Age</th></tr> <tr><td>Kiran</td><td>25</td></tr> <tr><td>Nithin</td><td>30</td></tr> </table> """ # Read table from HTML content tables = pd.read_html(StringIO(html_content)) print('Output DataFrame from HTML Table:') print(tables[0])
Running this code will produce the following output −
Output DataFrame from HTML Table:
Name | Age | |
---|---|---|
0 | Kiran | 25 |
1 | Nithin | 30 |
Example: Extracting a Specific HTML Table with attrs
It is possible to extract a specific table from multiple HTML tables by using the attrs parameter of the read_html() method. In the following example we will extract the data from an HTML table which contains the id="employment_info".
import pandas as pd from io import StringIO # Create a string representing HTML table html_content = """ <table> <tr><th>Name</th><th>Age</th></tr> <tr><td>Kiran</td><td>25</td></tr> <tr><td>Nithin</td><td>30</td></tr> </table> <table id="employment_info"> <tr><th>Role</th><th>Salary</th></tr> <tr><td>HR</td><td>40000</td></tr> <tr><td>Sr Manager</td><td>60000</td></tr> </table> """ # Read the table with specific attributes tables = pd.read_html(StringIO(html_content), attrs={"id": "employment_info"}) print('Output DataFrame from HTML Table:') print(tables[0])
The output of the above code is as follows −
Output DataFrame from HTML Table:
Role | Salary | |
---|---|---|
0 | HR | 40000 |
1 | Sr Manager | 60000 |
Example: Reading HTML Tables from a URL
You can read tables from a URL containing multiple tables using the read_html() method and you can also filter the a specific table using the match parameter.
import pandas as pd # Read tables from a URL url = "https://www.tutorialspoint.com/python_pandas/python_pandas_descriptive_statistics.htm" # Read the table matching "cumsum" tables = pd.read_html(url, match="cumsum", ) print('Output DataFrame from HTML Table:') print(tables[0])
The output of the above code contains the filtered data −
Output DataFrame from HTML Table:
Sr.No. | Methods & Description | |
---|---|---|
0 | 1 | cumsum() Return cumulative sum over a DataFrame... |
1 | 2 | cumprod() Return cumulative product over a Data... |
2 | 3 | cummax() Return cumulative maximum over a Data... |
3 | 4 | cummin() Return cumulative minimum over a Data... |
Example: Extracting Hyperlinks While Reading an HTML Table
This example demonstrates how to extract hyperlinks while reading an HTML table into Pandas DataFrame using the extract_links parameter of the read_html() method.
import pandas as pd from io import StringIO # Create a string representing HTML table html_content = """ <table border="1" class="dataframe"> <thead> <tr style="text-align: right;"> <th></th> <th>Name</th> <th>URL</th> </tr> </thead> <tbody> <tr> <th>0</th> <td>Tutorialspoint</td> <td><a href="https://www.tutorialspoint.com/index.htm" target="_blank">https://www.tutorialspoint.com/index.htm</a></td> </tr> <tr> <th>1</th> <td>Python Pandas Tutorial</td> <td><a href="https://www.tutorialspoint.com/python_pandas/index.htm" target="_blank">https://www.tutorialspoint.com/python_pandas/index.htm</a></td> </tr> </tbody> </table> """ # Extract hyperlinks from the HTML Table tables = pd.read_html(StringIO(html_content), extract_links="all") print('Output from reading HTML Table:') print(tables[0])
On executing the above code we will get the following output −
Output from reading HTML Table:
(, None) | ... | (URL, None) | |
---|---|---|---|
0 | (0, None) | ... | (https://www.tutorialspoint.com/index.htm, htt...) |
1 | (1, None) | ... | (https://www.tutorialspoint.com/python_pandas/... |