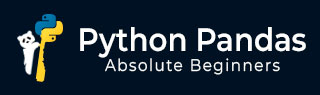
- Python Pandas - Home
- Python Pandas - Introduction
- Python Pandas - Environment Setup
- Python Pandas - Basics
- Python Pandas - Introduction to Data Structures
- Python Pandas - Index Objects
- Python Pandas - Panel
- Python Pandas - Basic Functionality
- Python Pandas - Indexing & Selecting Data
- Python Pandas - Series
- Python Pandas - Series
- Python Pandas - Slicing a Series Object
- Python Pandas - Attributes of a Series Object
- Python Pandas - Arithmetic Operations on Series Object
- Python Pandas - Converting Series to Other Objects
- Python Pandas - DataFrame
- Python Pandas - DataFrame
- Python Pandas - Accessing DataFrame
- Python Pandas - Slicing a DataFrame Object
- Python Pandas - Modifying DataFrame
- Python Pandas - Removing Rows from a DataFrame
- Python Pandas - Arithmetic Operations on DataFrame
- Python Pandas - IO Tools
- Python Pandas - IO Tools
- Python Pandas - Working with CSV Format
- Python Pandas - Reading & Writing JSON Files
- Python Pandas - Reading Data from an Excel File
- Python Pandas - Writing Data to Excel Files
- Python Pandas - Working with HTML Data
- Python Pandas - Clipboard
- Python Pandas - Working with HDF5 Format
- Python Pandas - Comparison with SQL
- Python Pandas - Data Handling
- Python Pandas - Sorting
- Python Pandas - Reindexing
- Python Pandas - Iteration
- Python Pandas - Concatenation
- Python Pandas - Statistical Functions
- Python Pandas - Descriptive Statistics
- Python Pandas - Working with Text Data
- Python Pandas - Function Application
- Python Pandas - Options & Customization
- Python Pandas - Window Functions
- Python Pandas - Aggregations
- Python Pandas - Merging/Joining
- Python Pandas - MultiIndex
- Python Pandas - Basics of MultiIndex
- Python Pandas - Indexing with MultiIndex
- Python Pandas - Advanced Reindexing with MultiIndex
- Python Pandas - Renaming MultiIndex Labels
- Python Pandas - Sorting a MultiIndex
- Python Pandas - Binary Operations
- Python Pandas - Binary Comparison Operations
- Python Pandas - Boolean Indexing
- Python Pandas - Boolean Masking
- Python Pandas - Data Reshaping & Pivoting
- Python Pandas - Pivoting
- Python Pandas - Stacking & Unstacking
- Python Pandas - Melting
- Python Pandas - Computing Dummy Variables
- Python Pandas - Categorical Data
- Python Pandas - Categorical Data
- Python Pandas - Ordering & Sorting Categorical Data
- Python Pandas - Comparing Categorical Data
- Python Pandas - Handling Missing Data
- Python Pandas - Missing Data
- Python Pandas - Filling Missing Data
- Python Pandas - Interpolation of Missing Values
- Python Pandas - Dropping Missing Data
- Python Pandas - Calculations with Missing Data
- Python Pandas - Handling Duplicates
- Python Pandas - Duplicated Data
- Python Pandas - Counting & Retrieving Unique Elements
- Python Pandas - Duplicated Labels
- Python Pandas - Grouping & Aggregation
- Python Pandas - GroupBy
- Python Pandas - Time-series Data
- Python Pandas - Date Functionality
- Python Pandas - Timedelta
- Python Pandas - Sparse Data Structures
- Python Pandas - Sparse Data
- Python Pandas - Visualization
- Python Pandas - Visualization
- Python Pandas - Additional Concepts
- Python Pandas - Caveats & Gotchas
Python Pandas read_clipboard() Method
The read_clipboard() method in Python's Pandas library provides an easy way to read data copied to the system clipboard and directly convert it into a Pandas DataFrame. This functionality is particularly useful for quickly importing tabular data from other sources such as Microsoft Excel, Google Sheets, or web pages.
This method initially reads the text from clipboard and pass it to the read_csv() method. Since read_clipboard() internally uses the read_csv() method, it supports many of the same arguments, making it a flexible tool for data parsing.
Syntax
Following is the syntax of the Python Pandas read_clipboard() method −
pandas.read_clipboard(sep='\\s+', dtype_backend=<no_default>, **kwargs)
Parameters
The read_clipboard() method accepts the following parameters −
sep: A string or regex delimiter that separates values. By default it is set to '\\s+', which denotes one or more whitespace characters.
dtype_backend: Determines the backend data type for the resultant DataFrame. Available options are numpy_nullable and pyarrow.
**kwargs: Additional arguments that can be passed to read_csv() for customization.
Return Value
The read_clipboard() method returns a DataFrame object containing the parsed clipboard content.
Example: Reading a DataFrame from Clipboard
The following example demonstrates how to copy a Pandas DataFrame to the clipboard and read it back using read_clipboard().
import pandas as pd # Creating a sample DataFrame df = pd.DataFrame([[1, 2, 3], [4, 5, 6]], columns=['A', 'B', 'C']) # Copy DataFrame to clipboard df.to_clipboard() # Read data from clipboard clipboard_df = pd.read_clipboard() # Display the DataFrame print('DataFrame from clipboard:') print(clipboard_df)
When we run above program, it produces following result −
DataFrame from clipboard:
A | B | C | |
---|---|---|---|
0 | 1 | 2 | 3 |
1 | 4 | 5 | 6 |
Example: Importing Clipboard Data with Custom Separator
If the copied data contains different delimiter other than comma(,), we can specify the separator by using the sep parameter of the read_clipboard() method.
Before executing the below code please copy the following data.
Index;A;B 0;10;30 1;20;40
import pandas as pd # Read clipboard data with tab separator clipboard_df = pd.read_clipboard(sep=';') # Display the DataFrame print('DataFrame from clipboard:') print(clipboard_df)
While executing the above code we get the following output −
DataFrame from clipboard:
Index | A | B | |
---|---|---|---|
0 | 0 | 10 | 30 |
1 | 1 | 20 | 40 |
Example: Reading Clipboard Data with Index
If the copied data contains row labels, Pandas will recognize them as an index and converts it into Pandas DataFame as it is.
Before executing the below code please copy the following data.
A B C x 1 4 p y 2 5 q z 3 6 r
import pandas as pd # Read clipboard data clipboard_df = pd.read_clipboard() # Display the DataFrame print('DataFrame from Clipboard:') print(clipboard_df)
Following is an output of the above code −
DataFrame from Clipboard:
A | B | C | |
---|---|---|---|
x | 1 | 4 | p |
y | 2 | 5 | q |
z | 3 | 6 | r |
Example: Reading Clipboard Data with Missing Values
If the clipboard data contains missing values, Pandas will automatically represent them as NaN when using the read_clipboard() method. The following example demonstrates how Pandas read_clipboard() method handles missing values in the copied data.
Before executing the below code please copy the following data.
ID Name Score 101 Kiara 85 102 Rajesh 103 Adhya 78 104 Saranya 92
import pandas as pd # Read the copied data from clipboard df = pd.read_clipboard(sep="\\s+") # Display the DataFrame print('DataFrame from Clipboard:') print(df)
Following is an output of the above code −
DataFrame from Clipboard:
ID | Name | Score | |
---|---|---|---|
0 | 101 | Kiara | 85.0 |
1 | 102 | Rajesh | NaN |
2 | 103 | Adhya | 78.0 |
3 | 104 | Saranya | 92.0 |