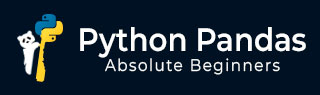
- Python Pandas - Home
- Python Pandas - Introduction
- Python Pandas - Environment Setup
- Python Pandas - Basics
- Python Pandas - Introduction to Data Structures
- Python Pandas - Index Objects
- Python Pandas - Panel
- Python Pandas - Basic Functionality
- Python Pandas - Indexing & Selecting Data
- Python Pandas - Series
- Python Pandas - Series
- Python Pandas - Slicing a Series Object
- Python Pandas - Attributes of a Series Object
- Python Pandas - Arithmetic Operations on Series Object
- Python Pandas - Converting Series to Other Objects
- Python Pandas - DataFrame
- Python Pandas - DataFrame
- Python Pandas - Accessing DataFrame
- Python Pandas - Slicing a DataFrame Object
- Python Pandas - Modifying DataFrame
- Python Pandas - Removing Rows from a DataFrame
- Python Pandas - Arithmetic Operations on DataFrame
- Python Pandas - IO Tools
- Python Pandas - IO Tools
- Python Pandas - Working with CSV Format
- Python Pandas - Reading & Writing JSON Files
- Python Pandas - Reading Data from an Excel File
- Python Pandas - Writing Data to Excel Files
- Python Pandas - Working with HTML Data
- Python Pandas - Clipboard
- Python Pandas - Working with HDF5 Format
- Python Pandas - Comparison with SQL
- Python Pandas - Data Handling
- Python Pandas - Sorting
- Python Pandas - Reindexing
- Python Pandas - Iteration
- Python Pandas - Concatenation
- Python Pandas - Statistical Functions
- Python Pandas - Descriptive Statistics
- Python Pandas - Working with Text Data
- Python Pandas - Function Application
- Python Pandas - Options & Customization
- Python Pandas - Window Functions
- Python Pandas - Aggregations
- Python Pandas - Merging/Joining
- Python Pandas - MultiIndex
- Python Pandas - Basics of MultiIndex
- Python Pandas - Indexing with MultiIndex
- Python Pandas - Advanced Reindexing with MultiIndex
- Python Pandas - Renaming MultiIndex Labels
- Python Pandas - Sorting a MultiIndex
- Python Pandas - Binary Operations
- Python Pandas - Binary Comparison Operations
- Python Pandas - Boolean Indexing
- Python Pandas - Boolean Masking
- Python Pandas - Data Reshaping & Pivoting
- Python Pandas - Pivoting
- Python Pandas - Stacking & Unstacking
- Python Pandas - Melting
- Python Pandas - Computing Dummy Variables
- Python Pandas - Categorical Data
- Python Pandas - Categorical Data
- Python Pandas - Ordering & Sorting Categorical Data
- Python Pandas - Comparing Categorical Data
- Python Pandas - Handling Missing Data
- Python Pandas - Missing Data
- Python Pandas - Filling Missing Data
- Python Pandas - Interpolation of Missing Values
- Python Pandas - Dropping Missing Data
- Python Pandas - Calculations with Missing Data
- Python Pandas - Handling Duplicates
- Python Pandas - Duplicated Data
- Python Pandas - Counting & Retrieving Unique Elements
- Python Pandas - Duplicated Labels
- Python Pandas - Grouping & Aggregation
- Python Pandas - GroupBy
- Python Pandas - Time-series Data
- Python Pandas - Date Functionality
- Python Pandas - Timedelta
- Python Pandas - Sparse Data Structures
- Python Pandas - Sparse Data
- Python Pandas - Visualization
- Python Pandas - Visualization
- Python Pandas - Additional Concepts
- Python Pandas - Caveats & Gotchas
Python Pandas - Parquet File Format
Parquet File Format in Pandas
The parquet file format in Pandas is binary columnar file format designed for efficient serialization and deserialization of Pandas DataFrames. It supports all Pandas data types, including extension types such as categorical and timezone-aware datetime types. The format is based on Apache Arrow's memory specification, enabling high-performance I/O operations.
Apache Parquet is a popular, open-source, column-oriented storage format designed for efficient reading and writing of DataFrames and can provide the options for easily sharing data across data analysis languages. It supports multiple compression methods to reduce file size while ensuring efficient reading performance.
Pandas provides robust support for Parquet file format, enabling efficient data serialization and de-serialization. In this tutorial, we will learn how to handle Parquet file format using Python's Pandas library.
Important Considerations
When working with parquet files in Pandas, you need to consider the following key points in mind −
Column Name Restrictions: Duplicate column name and non-string column names are not supported. If index level names are specified, they must also be strings.
Choosing an Engine: Supported engines include pyarrow, fastparquet, or auto. If no engine is specified, Pandas uses the pd.options.io.parquet.engine setting. If set to auto, Pandas tries to use pyarrow first and falls back to fastparquet if necessary.
Index Handling: pyarrow engine writes the index by default, while fastparquet only writes non-default indexes. This difference can cause issues for non-Pandas consumers. Use the index argument to control this explicitly.
Categorical Data Types: The pyarrow engine supports categorical data types, including the ordered flag for string categories. Whereas the fastparquet engine supports categorical types but does not preserve the ordered flag.
Unsupported Data Types: Data types like Interval and object types are not supported and will raise serialization errors.
Extension Data Types: The pyarrow engine preserves Pandas extension types like nullable integer and string data types (starting from pyarrow version 0.16.0). These types must implement the required protocols for serialization.
These considerations ensure that smooth data serialization and deserialization when working with Parquet files in Pandas.
Saving a Pandas DataFrame to a parquet File
To save a Pandas DataFrame to a parquet file, you can use the DataFrame.to_parquet() method, which saves data of the Pandas DataFrame to a file in parquet format.
Note: Before saving or retrieving the data from a parquet file you need to ensure that either the 'pyarrow' or 'fastparquet' libraries are installed. These are the optional Python dependency libraries that needs to be installed it by using the following commands −pip install pyarrow. pip install fastparquet
Example
This example shows how to save DataFrames to Parquet file format using the DataFrame.to_parquet() method, here we are saving it with the "pyarrow" engine.
import pandas as pd import numpy as np # Create a sample DataFrame df = pd.DataFrame({ "a": list("abc"), "b": list(range(1, 4)), "c": np.arange(3, 6).astype("u1"), "d": np.arange(4.0, 7.0), "e": [True, False, True], "f": pd.Categorical(list("abc")), "g": pd.date_range("20240101", periods=3) }) print("Original DataFrame:") print(df) # Save the DataFrame as a parquet file df.to_parquet("df_parquet_file.parquet", engine="pyarrow") print("\nDataFrame is successfully saved as a parquet file.")
When we run above program, it produces following result −
Original DataFrame:
a | b | c | d | e | f | g | |
---|---|---|---|---|---|---|---|
0 | a | 1 | 3 | 4.0 | True | a | 2024-01-01 |
1 | b | 2 | 4 | 5.0 | False | b | 2024-01-02 |
2 | c | 3 | 5 | 6.0 | True | c | 2024-01-01 |
If you visit the folder where the parquet files are saved, you can observe the generated parquet file.
Reading Data from a parquet File
For reading a parquet file data into the Pandas object, you can use the Pandas read_parquet() method. This method provides more options for reading parquet file from a variety of storage backends, including local files, URLs, and cloud storage services.
Example
This example reads the Pandas DataFrame from a parquet file using the Pandas read_parquet() method.
import pandas as pd import numpy as np # Create a sample DataFrame df = pd.DataFrame({ "a": list("abc"), "b": list(range(1, 4)), "c": np.arange(3, 6).astype("u1"), "d": np.arange(4.0, 7.0), "e": [True, False, True], "f": pd.Categorical(list("abc")), "g": pd.date_range("20240101", periods=3) }) # Save the DataFrame as a parquet file df.to_parquet("df_parquet_file.parquet") # Load the parquet file result = pd.read_parquet("df_parquet_file.parquet") # Display the DataFrame print('Loaded DataFrame:') print(result) # Verify data types print("\nData Type of the each column:") print(result.dtypes)
While executing the above code we get the following output −
Loaded DataFrame:
a | b | c | d | e | f | g | |
---|---|---|---|---|---|---|---|
0 | a | 1 | 3 | 4.0 | True | a | 2024-01-01 |
1 | b | 2 | 4 | 5.0 | False | b | 2024-01-02 |
2 | c | 3 | 5 | 6.0 | True | c | 2024-01-03 |
Reading and Writing Parquet Files In-Memory
You can also store and retrieve the parquet format data in Python in-memory. In-memory files store data in RAM instead of writing to disk, making them ideal for temporary data processing while avoiding file I/O operations. Python provides several types of in-memory files, here we will use the BytesIO for reading and write the parquet format data.
Example
This example demonstrates reading and writing a DataFrame as a parquet format In-Memory using the read_parquet() and DataFrame.to_parquet() methods with the help of the BytesIO library.
import pandas as pd import io # Create a DataFrame df = pd.DataFrame({"Col_1": range(5), "Col_2": range(5, 10)}) print("Original DataFrame:") print(df) # Save the DataFrame as In-Memory parquet buf = io.BytesIO() df.to_parquet(buf) # Read the DataFrame from the in-memory buffer loaded_df = pd.read_parquet(buf) print("\nDataFrame Loaded from In-Memory parquet:") print(loaded_df)
Following is an output of the above code −
Original DataFrame:
Col_1 | Col_2 | |
---|---|---|
0 | 0 | 5 |
1 | 1 | 6 |
2 | 2 | 7 |
3 | 3 | 8 |
4 | 4 | 9 |
Col_1 | Col_2 | |
---|---|---|
0 | 0 | 5 |
1 | 1 | 6 |
2 | 2 | 7 |
3 | 3 | 8 |
4 | 4 | 9 |