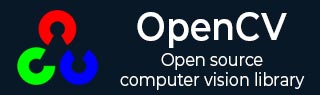
- OpenCV - Home
- OpenCV - Overview
- OpenCV - Environment
- OpenCV - Storing Images
- OpenCV - Reading Images
- OpenCV - Writing an Image
- OpenCV - GUI
- Image Conversion
- Colored Images to GrayScale
- OpenCV - Colored Image to Binary
- OpenCV - Grayscale to Binary
- Drawing Functions
- OpenCV - Drawing a Circle
- OpenCV - Drawing a Line
- OpenCV - Drawing a Rectangle
- OpenCV - Drawing an Ellipse
- OpenCV - Drawing Polylines
- OpenCV - Drawing Convex Polylines
- OpenCV - Drawing Arrowed Lines
- OpenCV - Adding Text
- Filtering
- OpenCV - Bilateral Filter
- OpenCV - Box Filter
- OpenCV - SQRBox Filter
- OpenCV - Filter2D
- OpenCV - Dilation
- OpenCV - Erosion
- OpenCV - Morphological Operations
- OpenCV - Image Pyramids
- Sobel Derivatives
- OpenCV - Sobel Operator
- OpenCV - Scharr Operator
- Transformation Operations
- OpenCV - Laplacian Transformation
- OpenCV - Distance Transformation
- Camera and Face Detection
- OpenCV - Using Camera
- OpenCV - Face Detection in a Picture
- Face Detection using Camera
- Geometric Transformations
- OpenCV - Affine Translation
- OpenCV - Rotation
- OpenCV - Scaling
- OpenCV - Color Maps
- Miscellaneous Chapters
- OpenCV - Canny Edge Detection
- OpenCV - Hough Line Transform
- OpenCV - Histogram Equalization
- OpenCV Useful Resources
- OpenCV - Quick Guide
- OpenCV - Cheatsheet
- OpenCV - Useful Resources
- OpenCV - Discussion
Python OpenCV Cheatsheet
The Python OpenCV cheatsheet provides the basic concepts for all its fundamental topics. OpenCV is an open-source computer vision library that allows computer programmers to process images or videos. By learning this cheat sheet, one can prepare for the interviews and exams. Go through this cheat sheet and learn the OpenCV.
Table of Content
- Basics and Installation
- Image Processing and Manipulation
- Geometric Transformations
- Drawing and Annotations
- Contours and Object Detection
- Feature Detection and Tracking
- Advanced Image Segmentation and Processing
- Machine Learning and Deep Learning in OpenCV
- 3D Vision and Depth Estimation
1. Basics and Installation
OpenCV is the computer vision library that processes the images and video.
i. Installing OpenCV
To install OpenCV on your system, use the following command −
pip install opencv-python
ii. Importing OpenCV
To import the OpenCV library, use the following line of code −
import cv2
iii. Reading and Displaying an Image
To read and display the images from the file, below are a few lines of code to understand its usage −
import cv2 # Read the image image = cv2.imread("image.jpg") # Show the image cv2.imshow("Image", image) # Wait for a key press cv2.waitKey(0) # Close the window cv2.destroyAllWindows()
iv. Writing and Saving an Image
In OpenCV, to write and save an image from a file, follow the below lines of code −
import cv2 # Read the image image = cv2.imread("example_image.jpg") # Save the image cv2.imwrite("example_output.jpg", image)
v. Reading and Displaying a Video
In OpenCV, we can read a video file and play it frame by frame.
import cv2 # Open video file cap = cv2.VideoCapture("video.mp4") while cap.isOpened(): ret, frame = cap.read() if not ret: break cv2.imshow("Video", frame) if cv2.waitKey(25) & 0xFF == ord("q"): break cap.release() cv2.destroyAllWindows()
vi. Capturing Video from Webcam
To capture video using a webcam and display it live, use the following steps of code −
import cv2 # Open the webcam cap = cv2.VideoCapture(0) while True: ret, frame = cap.read() if not ret: break cv2.imshow("Webcam", frame) if cv2.waitKey(1) & 0xFF == ord("q"): break cap.release() cv2.destroyAllWindows()
2. Image Processing and Manipulation
Image processing is the technique to improve the quality of an image or extract information.
i. Resizing an Image
We can resize an image to a specific size by specifying the width and height. The cv2.resize() function is used to perform this operation.
import cv2 # Load the image image = cv2.imread('image.jpg') # Resize the image to a specific size (width, height) resized_image = cv2.resize(image, (400, 300)) # Save the resized image cv2.imwrite('resized_image.jpg', resized_image)
ii. Converting Color Spaces
The color space define the conversion of an image from one color space to another. For references, you can convert a BGR image to grayscale using the cv2.cvtColor() function.
import cv2 # Load the image image = cv2.imread('image.jpg') # Convert the image to grayscale gray_image = cv2.cvtColor(image, cv2.COLOR_BGR2GRAY) # Save the grayscale image cv2.imwrite('gray_image.jpg', gray_image)
iii. Image Thresholding
Image thresholding is the process of segmenting an image into two regions based on pixel density.
import cv2 # Load the image in grayscale image = cv2.imread('example_image.jpg', cv2.IMREAD_GRAYSCALE) # Apply simple thresholding _, thresh_image = cv2.threshold(image, 127, 255, cv2.THRESH_BINARY) # Save the thresholded image cv2.imwrite('threshold_image.jpg', thresh_image) # Apply adaptive thresholding adaptive_thresh = cv2.adaptiveThreshold(image, 255, cv2.ADAPTIVE_THRESH_MEAN_C, cv2.THRESH_BINARY, 11, 2) # Save the adaptive threshold image cv2.imwrite('adaptive_thresh_image.jpg', adaptive_thresh)
iv. Blurring and Smoothing
To blur and smooth reduction noise from an image, use the function "cv2.GaussianBlur()" for the Gaussian filter and cv2.medianBlur() for the median filter.
import cv2 # Load the image image = cv2.imread('image.jpg') # Apply Gaussian Blur blurred_image = cv2.GaussianBlur(image, (5, 5), 0) # Save the blurred image cv2.imwrite('blurred_image.jpg', blurred_image) # Apply Median Blur median_blurred_image = cv2.medianBlur(image, 5) # Save the median blurred image cv2.imwrite('median_blurred_image.jpg', median_blurred_image)
v. Edge Detection
Edge detection is used to find boundaries from an image. The cv2.Canny() function is very common to use for detecting edges based on intensity gradients.
import cv2 # Load the image in grayscale image = cv2.imread('image.jpg', cv2.IMREAD_GRAYSCALE) # Apply Canny edge detection edges = cv2.Canny(image, 100, 200) # Save the edge-detected image cv2.imwrite('edges_image.jpg', edges)
vi. Bitwise Operations
In OpenCV, we can perform the bitwise operations such as AND, OR, and NOT that are used for masking and image combination. These operations change the individual pixels based on the binary values.
import cv2 import numpy as np # Load two images image1 = cv2.imread('image1.jpg') image2 = cv2.imread('image2.jpg') # Bitwise AND operation and_image = cv2.bitwise_and(image1, image2) # Bitwise OR operation or_image = cv2.bitwise_or(image1, image2) # Bitwise NOT operation not_image = cv2.bitwise_not(image1) # Save the result images cv2.imwrite('and_image.jpg', and_image) cv2.imwrite('or_image.jpg', or_image) cv2.imwrite('not_image.jpg', not_image)
vii. Image Histograms
In OpenCV, a histogram visualizes the distribution of the pixel intensities from an image. So, cv2.calcHist() calculates the histogram, and cv2.equalizeHist() enhances image contrast by adjusting the histogram.
import cv2 # Load the image in grayscale image = cv2.imread('image.jpg', cv2.IMREAD_GRAYSCALE) # Calculate the histogram of the image hist = cv2.calcHist([image], [0], None, [256], [0, 256]) # Equalize the histogram to improve the contrast equalized_image = cv2.equalizeHist(image) # Save the equalized image cv2.imwrite('equalized_image.jpg', equalized_image)
3. Geometric Transformations
In OpenCV, geometric transformation is the process of modifying the spatial properties of an image, such as position, size, or orientation.
i. Image Rotation
You can rotate an image from the center specified angle. Here, the cv2.getRotationMatrix2D() function creates a rotation matrix, while cv2.warpAffine() applies the rotation transformation.
import cv2 # Load the image image = cv2.imread('image.jpg') # Get the image dimensions height, width = image.shape[:2] # Get the rotation matrix center = (width / 2, height / 2) angle = 45 scale = 1.0 rotation_matrix = cv2.getRotationMatrix2D(center, angle, scale) # Apply the rotation to the image rotated_image = cv2.warpAffine(image, rotation_matrix, (width, height)) # Save the rotated image cv2.imwrite('rotated_image.jpg', rotated_image)
ii. Image Translation
Translate an image by shifting it along the x and y axes. The cv2.warpAffine() function can be used to apply a translation matrix to move the image.
import cv2 import numpy as np # Load the image image = cv2.imread('image.jpg') # Get the image dimensions height, width = image.shape[:2] # Define the translation matrix translation_matrix = np.float32([[1, 0, 100], [0, 1, 50]]) # Apply the translation of the image translated_image = cv2.warpAffine(image, translation_matrix, (width, height)) # Save the translated image cv2.imwrite('translated_image.jpg', translated_image)
iii. Image Scaling
An image scaling means resizing the images by a factor or specifying size. Use cv2.resize() to perform scaling.
import cv2 # Load the image image = cv2.imread('image.jpg') # Scale the image by a factor of 0.5 (50%) scaled_image = cv2.resize(image, None, fx=0.5, fy=0.5) # Save the scaled image cv2.imwrite('scaled_image.jpg', scaled_image)
iv. Perspective Transformation
Theperspective transformation is used to change the view of an image.
import cv2 import numpy as np # Load the image image = cv2.imread('image.jpg') # Define points for perspective transformation # Four points from the original image and their corresponding points in the transformed image pts1 = np.float32([[50, 50], [200, 50], [50, 200], [200, 200]]) pts2 = np.float32([[10, 100], [210, 50], [50, 250], [220, 210]]) # Get the perspective transformation matrix matrix = cv2.getPerspectiveTransform(pts1, pts2) # Apply the perspective transformation perspective_image = cv2.warpPerspective(image, matrix, (image.shape[1], image.shape[0])) # Save the transformed image cv2.imwrite('perspective_image.jpg', perspective_image)
4. Drawing and Annotations
The drawing and annotations define the process of adding information to an image.
i. Drawing Shapes
While drawing basic shapes on an image, such as lines, circles, rectangles, and polygons. Use the functions like cv2.line(), cv2.circle(), cv2.rectangle(), and cv2.polylines().
import cv2 import numpy as np # Create a blank white image image = np.ones((500, 500, 3), dtype=np.uint8) * 255 # Drawing a line cv2.line(image, (50, 50), (450, 450), (0, 0, 255), 5) # Drawing a circle cv2.circle(image, (250, 250), 100, (0, 255, 0), -1) # Drawing a rectangle cv2.rectangle(image, (100, 100), (400, 400), (255, 0, 0), 3) # Drawing a polygon (triangle) pts = np.array([[250, 50], [100, 400], [400, 400]], np.int32) pts = pts.reshape((-1, 1, 2)) cv2.polylines(image, [pts], isClosed=True, color=(255, 255, 0), thickness=4) # Save the image cv2.imwrite('shapes_image.jpg', image)
ii. Adding Text to an Image
To add the text from an image, use the function cv2.putText(). Also, you can specify the text font, size, color, and position of the text on the image.
import cv2 # Create a blank white image image = np.ones((500, 500, 3), dtype=np.uint8) * 255 # Add text to the image font = cv2.FONT_HERSHEY_SIMPLEX cv2.putText(image, 'Hello, OpenCV!', (100, 250), font, 1, (0, 0, 0), 2, cv2.LINE_AA) # Save the image with text cv2.imwrite('text_image.jpg', image)
5. Contours and Object Detection
The contour is a simple curve that joins all the continuous points.
i. Finding Contours
In OpenCV, contours are useful for detecting and analyzing objects in an image.
import cv2 import numpy as np # Load the image image = cv2.imread('image.jpg') # Convert the image to grayscale gray = cv2.cvtColor(image, cv2.COLOR_BGR2GRAY) # Apply thresholding to get binary image _, thresh = cv2.threshold(gray, 127, 255, cv2.THRESH_BINARY) # Find contours contours, _ = cv2.findContours(thresh, cv2.RETR_EXTERNAL, cv2.CHAIN_APPROX_SIMPLE) # Draw the contours on the image cv2.drawContours(image, contours, -1, (0, 255, 0), 3) # Green color, thickness = 3 # Save the image with contours cv2.imwrite('contours_image.jpg', image)
ii. Convex Hull and Contour Approximation
The convex hull is the smallest convex polygon that is surrounded by all the points of the contour. You can use the function like cv2.approxPolyDP() is used for approximating a polygonal curve.
import cv2 import numpy as np # Load the image image = cv2.imread('image.jpg') # Convert the image to grayscale gray = cv2.cvtColor(image, cv2.COLOR_BGR2GRAY) # Apply thresholding to get binary image _, thresh = cv2.threshold(gray, 127, 255, cv2.THRESH_BINARY) # Find contours contours, _ = cv2.findContours(thresh, cv2.RETR_EXTERNAL, cv2.CHAIN_APPROX_SIMPLE) # Get the convex hull for the first contour hull = cv2.convexHull(contours[0]) # Approximate the contour to a polygon epsilon = 0.02 * cv2.arcLength(contours[0], True) approx = cv2.approxPolyDP(contours[0], epsilon, True) # Draw the convex hull and the approximated polygon cv2.drawContours(image, [hull], 0, (255, 0, 0), 3) # Blue color for hull cv2.drawContours(image, [approx], 0, (0, 255, 0), 3) # Green color for approximation # Save the image cv2.imwrite('hull_approx_image.jpg', image)
iii. Hough Transform for Line and Circle Detection
The Hough transform is used for detecting lines and circles in an image. The cv2.HoughLines() is used for line detection, and cv2.HoughCircles() is used for circle detection.
import cv2 import numpy as np # Load the image image = cv2.imread('image.jpg') # Convert to grayscale gray = cv2.cvtColor(image, cv2.COLOR_BGR2GRAY) # Detecting lines using Hough Line Transform edges = cv2.Canny(gray, 50, 150, apertureSize=3) lines = cv2.HoughLines(edges, 1, np.pi / 180, 100) for line in lines: rho, theta = line[0] x1 = int(rho * np.cos(theta) + 1000 * (-np.sin(theta))) y1 = int(rho * np.sin(theta) + 1000 * (np.cos(theta))) x2 = int(rho * np.cos(theta) - 1000 * (-np.sin(theta))) y2 = int(rho * np.sin(theta) - 1000 * (np.cos(theta))) cv2.line(image, (x1, y1), (x2, y2), (0, 0, 255), 2) # Red color for lines # Detecting circles using Hough Circle Transform circles = cv2.HoughCircles(gray, cv2.HOUGH_GRADIENT, dp=1.2, minDist=30, param1=50, param2=30, minRadius=10, maxRadius=100) if circles is not None: circles = np.round(circles[0, :]).astype("int") for (x, y, r) in circles: # Green color for circles cv2.circle(image, (x, y), r, (0, 255, 0), 4) # Save the image with detected lines and circles cv2.imwrite('hough_transform_image.jpg', image)
iv. Object Detection with Haar Cascades
In OpenCV haar cascades are used for object detection, such as detecting faces or other objects in an image.
import cv2 # Load the image image = cv2.imread('image.jpg') # Load the pre-trained Haar Cascade Classifier for face detection face_cascade = cv2.CascadeClassifier(cv2.data.haarcascades + 'haarcascade_frontalface_default.xml') # Convert to grayscale gray = cv2.cvtColor(image, cv2.COLOR_BGR2GRAY) # Detect faces in the image faces = face_cascade.detectMultiScale(gray, 1.3, 5) # Draw rectangles around detected faces for (x, y, w, h) in faces: cv2.rectangle(image, (x, y), (x + w, y + h), (255, 0, 0), 2) # Blue color for rectangle # Save the image with detected faces cv2.imwrite('haar_cascade_faces.jpg', image)
v. Face Detection using OpenCV
Face detection can be done using the pre-trained Haar cascades or DNN-based models in OpenCV. It is often used for real-time face recognition.
import cv2 # Load the image image = cv2.imread('image.jpg') # Load the pre-trained Haar Cascade Classifier for face detection face_cascade = cv2.CascadeClassifier(cv2.data.haarcascades + 'haarcascade_frontalface_default.xml') # Convert to grayscale gray = cv2.cvtColor(image, cv2.COLOR_BGR2GRAY) # Detect faces in the image faces = face_cascade.detectMultiScale(gray, 1.1, 4) # Draw rectangles around detected faces for (x, y, w, h) in faces: # Blue color for rectangle cv2.rectangle(image, (x, y), (x + w, y + h), (255, 0, 0), 2) # Save the image with detected faces cv2.imwrite('face_detection.jpg', image)
6. Feature Detection and Tracking
Feature detection and tracking are the processes to identify and follow objects in images or videos.
i. Corner Detection
In OpenCV, corner detection is used to identify the image where there is a significant change in the image gradient.
import cv2 import numpy as np # Load the image image = cv2.imread('image.jpg') # Convert to grayscale gray = cv2.cvtColor(image, cv2.COLOR_BGR2GRAY) # Detect corners using goodFeaturesToTrack corners = cv2.goodFeaturesToTrack(gray, 100, 0.01, 10) # Convert corners to integer corners = np.int0(corners) # Draw corners on the image for corner in corners: x, y = corner.ravel() cv2.circle(image, (x, y), 3, 255, -1) # Save the image with corners cv2.imwrite('corners_image.jpg', image)
ii. Feature Detection (ORB, SIFT, SURF)
Feature detection methods such as ORB, SIFT, and SURF are used to detect key points and features in an image.
import cv2 # Loading image image = cv2.imread('image.jpg') # Convert to grayscale gray = cv2.cvtColor(image, cv2.COLOR_BGR2GRAY) # Initialize ORB detector orb = cv2.ORB_create() # Detect keypoints and descriptors using ORB keypoints, descriptors = orb.detectAndCompute(gray, None) # Draw keypoints on the image image_with_keypoints = cv2.drawKeypoints(image, keypoints, None, (0, 255, 0), flags=cv2.DrawMatchesFlags_DRAW_RICH_KEYPOINTS) # Save the image with keypoints cv2.imwrite('orb_keypoints.jpg', image_with_keypoints)
iii. Optical Flow
Optical flow tracks object motion between two frames using movement patterns.
import cv2 import numpy as np # Load the image sequence (two consecutive frames) frame1 = cv2.imread('frame1.jpg') frame2 = cv2.imread('frame2.jpg') # Convert to grayscale gray1 = cv2.cvtColor(frame1, cv2.COLOR_BGR2GRAY) gray2 = cv2.cvtColor(frame2, cv2.COLOR_BGR2GRAY) # Calculate optical flow flow = cv2.calcOpticalFlowFarneback(gray1, gray2, None, 0.5, 3, 15, 3, 5, 1.2, 0) # Visualize the optical flow hsv = np.zeros_like(frame1) hsv[..., 1] = 255 mag, ang = cv2.cartToPolar(flow[..., 0], flow[..., 1]) hsv[..., 0] = ang * 180 / np.pi / 2 hsv[..., 2] = cv2.normalize(mag, None, 0, 255, cv2.NORM_MINMAX) flow_rgb = cv2.cvtColor(hsv, cv2.COLOR_HSV2BGR) # Save the optical flow image cv2.imwrite('optical_flow.jpg', flow_rgb)
iv. Real-time Object Tracking
Real−time object tracking methods such as CSRT and KCF are used to track a selected object across frames in a video stream.
import cv2 # Load video cap = cv2.VideoCapture('video.mp4') # Initialize the tracker tracker = cv2.TrackerCSRT_create() # Read the first frame and select the region of interest (ROI) for tracking ret, frame = cap.read() bbox = cv2.selectROI("Tracking", frame, fromCenter=False, showCrosshair=True) tracker.init(frame, bbox) while True: ret, frame = cap.read() if not ret: break # Update tracker and get the new bounding box ret, bbox = tracker.update(frame) # Draw bounding box if ret: p1 = (int(bbox[0]), int(bbox[1])) p2 = (int(bbox[0] + bbox[2]), int(bbox[1] + bbox[3])) cv2.rectangle(frame, p1, p2, (0, 255, 0), 2) else: cv2.putText(frame, "Tracking Failed", (100, 80), cv2.FONT_HERSHEY_SIMPLEX, 0.7, (0, 0, 255), 2) # Display the result cv2.imshow("Tracking", frame) # Exit if 'q' is pressed if cv2.waitKey(1) & 0xFF == ord('q'): break cap.release() cv2.destroyAllWindows()
7. Advanced Image Segmentation and Processing
Image segmentation is the computer vision technique that divides the images into separate regions or similar pixels.
i. Watershed Algorithm
The watershed algorithm segments images by flooding pixel topography.
import cv2 import numpy as np # Load the image image = cv2.imread('image.jpg') # Convert to grayscale gray = cv2.cvtColor(image, cv2.COLOR_BGR2GRAY) # Thresholding to get binary image _, thresh = cv2.threshold(gray, 0, 255, cv2.THRESH_BINARY_INV + cv2.THRESH_OTSU) # Applying distance transform and normalizing dist_transform = cv2.distanceTransform(thresh, cv2.DIST_L2, 5) _, sure_fg = cv2.threshold(dist_transform, 0.7 * dist_transform.max(), 255, 0) # Applying watershed sure_bg = cv2.dilate(thresh, None, iterations=3) unknown = cv2.subtract(sure_bg, sure_fg) markers = np.zeros_like(gray) markers[sure_fg == 255] = 1 markers[sure_bg == 255] = 2 cv2.watershed(image, markers) image[markers == -1] = [0, 0, 255] # Save the segmented image cv2.imwrite('watershed_image.jpg', image)
ii. GrabCut Algorithm
The GrabCut algorithm separates the foreground from the background. It uses a graph-based model and works iteratively for better segmentation.
import cv2 import numpy as np # Load the image image = cv2.imread('image.jpg') # Create an initial mask mask = np.zeros(image.shape[:2], np.uint8) # Define the foreground and background models bgd_model = np.zeros((1, 65), np.float64) fgd_model = np.zeros((1, 65), np.float64) # Define the rectangle that contains the foreground object rect = (50, 50, 450, 290) # Apply the GrabCut algorithm cv2.grabCut(image, mask, rect, bgd_model, fgd_model, 5, cv2.GC_INIT_WITH_RECT) # Modify the mask mask2 = np.where((mask == 2) | (mask == 0), 0, 1).astype('uint8') # Save the segmented image image = image * mask2[:, :, np.newaxis] cv2.imwrite('grabcut_image.jpg', image)
iii. Background Subtraction
Background subtraction detects motion by removing the background from a video frame.
import cv2 # Create background subtractor fgbg = cv2.createBackgroundSubtractorMOG2() # Open video cap = cv2.VideoCapture('video.mp4') while True: ret, frame = cap.read() if not ret: break # Apply background subtractor fgmask = fgbg.apply(frame) # Display the result cv2.imshow('Foreground Mask', fgmask) # Exit if 'q' is pressed if cv2.waitKey(1) & 0xFF == ord('q'): break cap.release() cv2.destroyAllWindows()
8. Machine Learning and Deep Learning in OpenCV
Below are the two points that demonstrate the model explaination in short −
i. Using Pre-trained DNN Models
In OpenCV, DNN Models is pre-trained that allows user to use deep learning model for various tasks such as object detection, classification, and segmentation.
import cv2 # Load a pre-trained deep learning model net = cv2.dnn.readNetFromCaffe('deploy.prototxt', 'mobilenet.caffemodel') # Load image and prepare for prediction image = cv2.imread('image.jpg') blob = cv2.dnn.blobFromImage(image, 1, (224, 224), (104, 117, 123)) # Perform forward pass and get predictions net.setInput(blob) predictions = net.forward() # Save the prediction result cv2.imwrite('dnn_prediction.jpg', image)
ii. Handwritten Digit Recognition
Handwritten digit recognition can be done using machine learning models such as SVM, KNN, or deep learning models on datasets like MNIST.
import cv2 import numpy as np from sklearn import datasets, svm # Load the MNIST dataset (using sklearn for simplicity) digits = datasets.load_digits() # Use SVM for handwritten digit recognition clf = svm.SVC(gamma=0.001, C=100) clf.fit(digits.data, digits.target) # Test with a sample image sample = digits.images[0] predicted_digit = clf.predict([sample.flatten()]) # Save the result print(f'Predicted digit: {predicted_digit}')
9. 3D Vision and Depth Estimation
3D vision estimates depth by calculating distances from a 2D image.
i. Pose Estimation (cv2.solvePnP())
Pose estimation is the process of determining the position and orientation of a 3D object in space, based on a set of 2D image points and corresponding 3D object points.
import cv2 import numpy as np # Define 3D object points (e.g., coordinates of the object in 3D space) object_points = np.array([[0, 0, 0], [1, 0, 0], [1, 1, 0], [0, 1, 0]], dtype=np.float32) # Define corresponding 2D image points image_points = np.array([[200, 300], [300, 300], [300, 400], [200, 400]], dtype=np.float32) # Camera matrix (intrinsic parameters of the camera) focal_length = 1 center = (0, 0) camera_matrix = np.array([[focal_length, 0, center[0]], [0, focal_length, center[1]], [0, 0, 1]]) # Distortion coefficients (no distortion in this case) dist_coeffs = np.zeros((4, 1)) # Solve for the rotation and translation vectors using solvePnP success, rotation_vector, translation_vector = cv2.solvePnP(object_points, image_points, camera_matrix, dist_coeffs) # Print the results print("Rotation Vector: \n", rotation_vector) print("Translation Vector: \n", translation_vector)
ii. Stereo Vision and Depth Mapping
Stereo vision uses two cameras to capture images from different angles and calculates their differences to estimate depth.
import cv2 import numpy as np # Load left and right stereo images left_image = cv2.imread('left_image.jpg', 0) right_image = cv2.imread('right_image.jpg', 0) # Create a stereo block matching object stereo = cv2.StereoBM_create(numDisparities=16, blockSize=15) # Compute disparity map disparity = stereo.compute(left_image, right_image) # Normalize the disparity map for better visualization disparity_normalized = cv2.normalize(disparity, None, 0, 255, cv2.NORM_MINMAX) # Display the disparity map cv2.imshow("Disparity Map", disparity_normalized) cv2.waitKey(0) cv2.destroyAllWindows()
iii. Image Stitching
The image stitching is the process to merge multiple images into a single, wider image with an extended field of view.
import cv2 import numpy as np # Load images to be stitched images = [cv2.imread('image1.jpg'), cv2.imread('image2.jpg'), cv2.imread('image3.jpg')] # Create a Stitcher object stitcher = cv2.createStitcher() # Perform the stitching status, stitched_image = stitcher.stitch(images) if status == cv2.Stitcher_OK: # Display the stitched panorama cv2.imshow("Panorama", stitched_image) cv2.waitKey(0) else: print("Error during stitching, status code:", status)