Development and validation of multi-sequence magnetic resonance imaging radiomics models for predicting tumor response to radiotherapy in locally advanced non-small cell lung cancer
Highlight box
Key findings
• In patients with locally advanced non-small cell lung cancer (NSCLC), the tumor response to radiotherapy can be predicted through the construction of magnetic resonance imaging (MRI) radiomics models.
• The predictive performance, clinical utility, and accuracy of the MRI combination sequence prediction model were found to be optimal, and thus model may provide valuable support for clinical research and treatment.
What is known and what is new?
• Significant progress has been made in the field of radiomics concerning lung cancer research. The primary focus has been on computed tomography (CT) and positron emission tomography-CT radiomics, which offer new possibilities for the precise diagnosis and treatment of lung cancer.
• This study pioneered the development of an MRI radiomics model for predicting tumor response to radiotherapy in patients with locally advanced NSCLC. The findings highlight the advantages and potential of MRI radiomics within the field of lung cancer.
What is the implication, and what should change now?
• This study aimed to enhance the exploration and application of MRI radiomics in various facets of lung cancer and ultimately yielded robust research conclusions. Furthermore, it sought to investigate and validate the potential value of MRI radiomics in predicting lung cancer outcomes.
Introduction
According to the latest data released by the International Agency for Research on Cancer, lung cancer remains the leading cause of death from malignant tumors worldwide (1). Among lung cancer cases, non-small cell lung cancer (NSCLC) accounts for approximately 85% of cases, with one-third of patients diagnosed at a locally advanced stage (stage III) (2). In the recent years, immunotherapies and targeted therapies have revolutionized the management of NSCLC (3-5). The application of sequential immunotherapy following concurrent chemoradiotherapy has significantly improved the survival outcomes of patients with locally advanced non-small cell lung cancer (LA-NSCLC) (6). However, more than 50% of patients still fail to achieve long-term survival (7). Over the past decade, with the continuous advancement of radiotherapy techniques, numerous studies have demonstrated the efficacy and safety of radiotherapy in patients with LA-NSCLC (8-10). Radiotherapy has become the standard treatment modality for unresectable stage III NSCLC.
Currently, there is a lack of effective models to predict tumor treatment response prior to radiotherapy in patients with highly heterogeneous LA-NSCLC. In recent years, various biomarker-based predictive models for NSCLC tumor response have been proposed, but their detection and interpretation are complex and costly, often requiring invasive procedures and stringent pathological tissue requirements. Additionally, some genes exhibit low mutation rates in NSCLC patients, leading to significant individual variations and limiting their routine application in the general population (11,12). Recently, studies have suggested that liquid biopsies, such as circulating tumor DNA (ctDNA) and certain protein biomarkers, can also monitor NSCLC treatment responses. However, limitations exist, including instances where baseline ctDNA levels are below detectable thresholds and the non-specificity of some biomarkers for cancer (13,14). Therefore, there is an urgent need to establish a noninvasive, comprehensive prediction model that accommodates individual variations. Radiomics, a novel noninvasive technology, enables the extraction of numerous quantitative parameters from imaging information that are invisible to the naked eye. This capability enables physicians to make more informed and precise diagnoses and treatment decisions (15). Mattonen et al. (16) analyzed the value of quantitative imaging features in assessing responses following radiotherapy for lung cancer. Recent studies have used computed tomography (CT) and positron emission tomography-computed tomography (PET-CT) for radiomics-based evaluation of tumor responses (17,18). A study found that the area under the curve (AUC) for any clinical endpoint in external validation of PET-CT radiomics models did not exceed 0.55 (19). Furthermore, some researchers reported negative results when validating CT radiomics models (20). This indicates that clinical decision-making and risk stratification based on CT or PET/CT radiomic features still face challenges in lung cancer diagnosis and management. The possible reasons for this may be that both imaging modalities rely on single-dimensional image analysis, and CT scans are prone to misinterpret certain benign manifestations of lung cancer (such as inflammation, atelectasis, necrosis, and fibrosis) as tumors. Furthermore, the heterogeneity within the dataset may also contribute to suboptimal predictive performance (18,21). However, magnetic resonance imaging (MRI), a widely used imaging technique, has yet to be thoroughly explored for its research potential in the field of lung cancer. MRI offers the advantages of multi-sequence and multi-parameter imaging, enabling it to investigate microstructural characteristics and physiological functions, it effectively compensates for the limitations associated with single-image modalities such as CT and PET-CT. Specifically, a study has indicated that one of these functional sequences, diffusion-weighted imaging (DWI), can provide unique information regarding the movement of water molecules, effectively distinguishing between central-type lung cancer and the boundaries of atelectasis (22). Mahdavi Rashed et al. (23) explored the application value of DWI in the diagnosis of pulmonary lesions. Their findings revealed a highly significant statistical difference in DWI grading between malignant and benign lesions on images with a b-value of 800 s/mm2 (P<0.001). Furthermore, MRI provides more comprehensive information in the assessment of tumor microenvironments (24,25). Through techniques such as metabolic imaging, the biological characteristics and activity of tumors can be analyzed. Jagoda et al. (26) found that DWI improved the assessment of treatment response in patients with NSCLC following radical radiotherapy and that radiation-induced pulmonary toxicity could also be accurately evaluated using MRI. This further underscores the unique value of MRI in monitoring radiotherapy for lung cancer.
Compared to CT and PET-CT, MRI offers excellent soft-tissue contrast at high spatial resolution (27). Some researchers have investigated the use of MRI for lung cancer screening, comparing its applicability to low-dose computed tomography (LDCT) in high-risk populations. The study revealed that MRI had a higher early recall rate compared to LDCT (13.8% vs. 12.5%) and demonstrated greater sensitivity and specificity in detecting nodules ≥6 mm (28). Furthermore, CT and PET-CT examinations typically involve radiation exposure, whereas MRI employs a non-radiative approach, making it more suitable for younger patients or cases requiring long-term follow-up.
Therefore, in light of the multiple advantages of MRI in the application to lung cancer, our objective is to develop and validate a machine learning model based on multi-sequence MRI radiomic features, with the aim of predicting the response of LA-NSCLC tumors to radiotherapy. We present this article in accordance with the TRIPOD reporting checklist (available at https://jtd.amegroups.com/article/view/10.21037/jtd-2025-142/rc).
Methods
The study was conducted in accordance with the Declaration of Helsinki (as revised in 2013). The study was approved by the Ethics Committee of Harbin Medical University Cancer Hospital (No. KY2023-05) and individual consent for this retrospective analysis was waived.
Population and tumor regression assessment
Data were retrospectively collected from all consecutive patients with NSCLC who underwent radiotherapy and pretreatment MRI scanning at the Harbin Medical University Cancer Hospital between November 2018 and April 2024.
The inclusion criteria were as follows: (I) age ≥18 years; (II) histologically confirmed NSCLC; (III) stage IIIA-IIIc NSCLC according to the eighth edition of the International Association for the Study of Lung Cancer (IASLC) lung cancer staging system (29); (IV) MR images obtained before radiotherapy, with the interval between MRI scanning and the start of radiotherapy not exceeding 2 weeks; and (V) comprehensive pretreatment MR images, including T1-weighted imaging (T1WI), T2-weighted imaging (T2WI), and DWI (b=800 s/mm²). The exclusion criteria included the following: (I) presence of stents that compromised MRI image quality or instances in which MRI exhibited motion artifacts, blurriness, or discontinuity; (II) lack of complete clinical follow-up data; and (III) radiation dose <5,000 cGy. Using a random sampling method, the patients were divided into a training set and a testing set at a ratio of 7:3. The training set and the testing set samples are utilized for model training and performance evaluation, respectively. Retrieve all cases and collect baseline clinical information of participants, including age, gender, pathological type, smoking status, performance status (PS) score, radiation dose, neutrophil-to-lymphocyte ratio (NLR), platelet-to-lymphocyte ratio (PLR), T stage, N stage, TNM staging, whether concurrent chemotherapy was administered, and whether immunotherapy was used.
The tumor regression was assessed according to the Response Evaluation Criteria in Solid Tumors (RECIST) version 1.1 (30). Lesion size changes were measured twice: once before radiotherapy and 1 month after radiotherapy. The RECIST criteria encompass several evaluation categories: complete response (CR), partial response (PR), stable disease (SD), and progressive disease (PD). In this study, CR and PR were classified as tumor regression sensitive (RS), while SD and PD were categorized as tumor regression resistant (RR) (21).
MRI images acquisition
Scans were performed using a 3.0-T Ingenia MRI system (Philips Healthcare, Best, the Netherlands). The patient was placed in a supine position, and DWI (b=800 s/mm2) was performed following T1WI and T2WI in order to determine the cross-sectional location for the DWI. Imaging was conducted using respiratory triggering techniques: a navigation band was placed between the lungs and the top of the liver, and end-expiration served as the trigger point (the starting point for scanning). MRI signal acquisition commenced at this point and continued until just before the following inhalation, which could minimize motion artifacts caused by respiration. The details of the parameters are provided in Table 1.
Table 1
Scanning sequence | Repetitive time (ms) | Reverberation time (ms) | Matrix | Perspective |
---|---|---|---|---|
T1WI | 4.8 | 1.4 | 300×300 | 450 mm × 450 mm |
T2WI | 814 | 70 | 252×178 | 380 mm × 319 mm |
DWI | 2,415 | 70 | 100×115 | 300 mm × 357 mm |
DWI, diffusion-weighted imaging; T1WI, T1-weighted imaging; T2WI, T2-weighted imaging.
Tumor delineation and segmentation
Two experts in radiation oncology with 5 years of working experience used ITK-SNAP software (www.itk-snap.org) to delineate the regions of interest (ROIs) along the margins of primary lung cancer lesions on axial images from T1WI, T2WI, and DWI. Subsequently, they manually traced the volumes of interest (VOIs) layer by layer (31). The overlapping regions delineated by these two physicians were subjected to radiomics analysis. A schematic representation of the ROI delineation is illustrated in Figure 1. To standardize the images from different individuals into a common spatial coordinate system for interindividual comparisons, we performed spatial normalization on the MR images using a fixed-resolution (1 mm × 1 mm × 1 mm) resampling method as part of the image preprocessing process (32). Finally, the z-score method was employed to standardize the data.
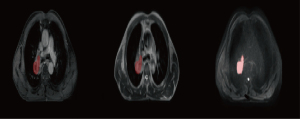
Feature extraction
All radiomic features were extracted using the internal feature analysis program implemented in Pyradiomics (http://pyradiomics.readthedocs.io). For each MRI sequence, seven feature groups were extracted: shape, first-order statistics, and texture features. The texture features included gray level size zone matrix (GLSZM), gray level co-occurrence matrix (GLCM), neighbouring gray tone difference matrix (NGTDM), gray level dependence matrix (GLDM), and gray-level run length matrix (GLRLM) (33). A total of 1,015 features were extracted from each of the T1WI, T2WI, and DWI sequences. Each patient had a cumulative total of 3,045 features extracted.
Radiomics features selection
The t-test was conducted for all radiomic features, followed by feature selection. Only those radiomic features with a P value <0.05 were retained. For features with high redundancy, the Pearson rank correlation coefficient was employed to assess the correlations between features. Among any pair of features exhibiting a correlation coefficient greater than 0.9, one feature was retained. To maximize the retention of descriptive capability regarding the features, a greedy recursive elimination strategy was implemented for feature filtering; specifically, this involved removing the feature that exhibited the highest redundancy in each iteration (34). The maximum relevance minimum redundancy (mRMR) method was then employed to select a subset of features that were highly correlated with the target variable and exhibited minimal redundancy among themselves. Finally, feature dimensionality reduction was performed using the least absolute shrinkage and selection operator (LASSO) regression model (35). The Python “scikit-learn” package is used for LASSO regression modeling, and the model parameters were optimized using ten-fold cross-validation. Given the limited number of patients in this study, it was essential to maximize the predictive performance of the model while minimizing overfitting. Therefore, the number of features was restricted to approximately 10% of the sample size. Consequently, in this study, each sequence was filtered to include no more than nine features.
Construction of radiomics models for individual and combined sequences
After the LASSO feature selection, we input the features selected from three individual sequences and the combined sequence into various machine learning models, including univariate logistic regression (LR), support vector machine (SVM), k-nearest neighbors (KNN), and random forest. This approach aimed to establish a radiomics model for predicting treatment response in patients with LA-NSCLC following radiotherapy.
Statistical analysis
The statistical analysis of clinical baseline characteristics was conducted using the Python “statsmodels” package (version 0.13.2). Continuous variables were analyzed using analysis of variance (ANOVA), while discrete variables were assessed with the Chi-squared (χ2) test to evaluate the clinical features of the patients (36). P value <0.05 indicated a statistically significant difference. The diagnostic efficacy of different radiomics models was evaluated using various assessment metrics, including the AUC, accuracy, sensitivity, and specificity. Subsequently, the optimal predictive model was selected. The predictive performance and clinical utility of this model were further assessed through calibration curves and decision curve analysis (DCA) (37).
Results
Population
A total of 137 patients with NSCLC and pretreatment MRI were identified through our database. Among them, 51 patients were excluded, 36 did not meet eligibility criteria (stage I–II–IV NSCLC), and 15 presented exclusion criteria (7 received a radiation dose <5,000 cGy, 7 had incomplete clinical information, and 1 had a poor image quality). A total of 86 patients were included in the final analysis (Figure 2: flowchart).
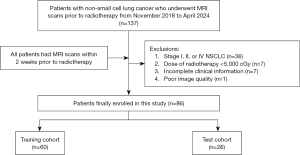
Specifically, 60 patients were assigned to the training set, while 26 patients were allocated to the test set. There were no significant differences in clinical factors between the RS group and the RR group in either the training set or test set (P>0.05). The basic clinical data of patients in both sets, along with details of their comparison, are presented in Table 2.
Table 2
Characteristic | Training set (n=60) | Test set (n=26) | |||||
---|---|---|---|---|---|---|---|
RS (n=32) | RR (n=28) | P | RS (n=14) | RR (n=12) | P | ||
Age (years) | 60.47±9.73 | 62.36±6.19 | 0.64 | 59.50±11.43 | 61.42±7.91 | 0.63 | |
Dose (cGy) | 6,107.50±297.29 | 6,060.36±371.87 | 0.79 | 5,956.43±437.86 | 6,136.67±164.45 | 0.34 | |
NLR | 2.96±2.40 | 3.31±4.48 | 0.54 | 2.84±1.95 | 2.45±1.04 | >0.99 | |
PLR | 191.07±183.77 | 155.06±107.10 | 0.22 | 152.42±63.26 | 119.76±40.30 | 0.14 | |
Sex | 0.14 | >0.99 | |||||
Male | 21 (65.62) | 24 (85.71) | 9 (64.29) | 7 (58.33) | |||
Female | 11 (34.38) | 4 (14.29) | 5 (35.71) | 5 (41.67) | |||
Histology | 0.72 | >0.99 | |||||
AD | 8 (25.00) | 5 (17.86) | 4 (28.57) | 4 (33.33) | |||
SCC | 24 (75.00) | 23 (82.14) | 10 (71.43) | 8 (66.67) | |||
Smoke | 0.85 | 0.98 | |||||
No | 13 (40.62) | 13 (46.43) | 7 (50.00) | 7 (58.33) | |||
Yes | 19 (59.38) | 15 (53.57) | 7 (50.00) | 5 (41.67) | |||
PS | 0.45 | 0.27 | |||||
0 | 8 (25.00) | 7 (25.00) | 5 (35.71) | 3 (25.00) | |||
1 | 20 (62.50) | 20 (71.43) | 7 (50.00) | 9 (75.00) | |||
2 | 4 (12.50) | 1 (3.57) | 2 (14.29) | 0 | |||
T stage | 0.49 | 0.68 | |||||
T1 | 1 (3.12) | 3 (10.71) | 1 (7.14) | 1 (8.33) | |||
T2 | 10 (31.25) | 11 (39.29) | 5 (35.71) | 7 (58.33) | |||
T3 | 15 (46.88) | 11 (39.29) | 4 (28.57) | 2 (16.67) | |||
T4 | 6 (18.75) | 3 (10.71) | 4 (28.57) | 2 (16.67) | |||
N stage | 0.47 | 0.54 | |||||
N0 | 0 | 2 (7.14) | 0 | 0 | |||
N1 | 2 (6.25) | 2 (7.14) | 1 (7.14) | 0 | |||
N2 | 20 (62.50) | 15 (53.57) | 9 (64.29) | 7 (58.33) | |||
N3 | 10 (31.25) | 9 (32.14) | 4 (28.57) | 5 (41.67) | |||
Disease stage | 0.20 | 0.42 | |||||
IIIA | 8 (25.00) | 13 (46.43) | 5 (35.71) | 6 (50.00) | |||
IIIB | 19 (59.38) | 11 (39.29) | 7 (50.00) | 3 (25.00) | |||
IIIC | 5 (15.62) | 4 (14.29) | 2 (14.29) | 3 (25.00) | |||
Chemotherapy | 0.51 | 0.12 | |||||
Sequential | 18 (56.25) | 19 (67.86) | 8 (57.14) | 11 (91.67) | |||
Concurrent | 14 (43.75) | 9 (32.14) | 6 (42.86) | 1 (8.33) | |||
Immunotherapy | >0.99 | 0.26 | |||||
Yes | 10 (31.25) | 8 (28.57) | 2 (14.29) | 5 (41.67) | |||
No | 22 (68.75) | 20 (71.43) | 12 (85.71) | 7 (58.33) |
Data are presented as n (%) or mean ± standard deviation. P value statistical methods: continuous variables are analyzed using ANOVA, while discrete variables are assessed through χ2 test. The content in parentheses represents the percentage of frequencies for discrete variables observed across different groups. ANOVA, analysis of variance; RS, regression sensitive; RR, regression resistant; NLR, neutrophil-to-lymphocyte ratio; PLR, platelet-to-lymphocyte ratio; AD, adenocarcinoma of lung; SCC, squamous cell carcinoma; PS, performance status.
Feature selection
From the T1WI, T2WI, and DWI sequences, 1,015 features were individually extracted. Subsequently, feature selection was performed for each sequence, resulting in the identification of four optimal features from T1WI, eight from T2WI, and six from DWI. The three sequence features were combined to obtain 3,045 combined sequence features. The features of the combined sequence were screened, and nine optimal radiomics features were finally selected, as shown in Figure 3.
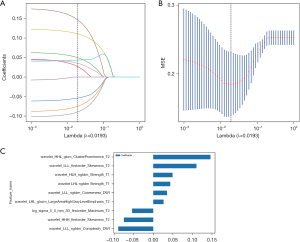
Model evaluation
The radiomic models were constructed based on the imaging features of each individual sequence and the combined sequences, which was followed by an evaluation of each model’s performance in both the training and test cohorts. Table 3 presents the diagnostic efficacy statistics of four machine learning models developed from individual and combined sequences within the training and test datasets.
Table 3
Sequence | Model name | Accuracy (%) | AUC (95% CI) | Sensitivity (%) | Specificity (%) | Cohort |
---|---|---|---|---|---|---|
T1WI | LR | 68.3 | 0.701 (0.564–0.838) | 53.6 | 81.2 | Train |
LR | 65.4 | 0.601 (0.365–0.837) | 66.7 | 64.3 | Test | |
SVM | 78.3 | 0.830 (0.722–0.939) | 82.1 | 75.0 | Train | |
SVM | 65.4 | 0.679 (0.467–0.890) | 33.3 | 92.9 | Test | |
KNN | 68.3 | 0.777 (0.663–0.890) | 32.1 | 100 | Train | |
KNN | 50.0 | 0.568 (0.354–0.783) | 25.0 | 71.4 | Test | |
Random forest | 86.7 | 0.944 (0.892–0.995) | 89.3 | 84.4 | Train | |
Random forest | 57.7 | 0.533 (0.296–0.770) | 83.3 | 35.7 | Test | |
T2WI | LR | 78.3 | 0.873 (0.784–0.961) | 92.9 | 65.6 | Train |
LR | 76.9 | 0.792 (0.606–0.977) | 91.7 | 64.3 | Test | |
SVM | 85.0 | 0.917 (0.837–0.998) | 89.3 | 81.2 | Train | |
SVM | 76.9 | 0.774 (0.580–0.967) | 83.3 | 71.4 | Test | |
KNN | 71.7 | 0.856 (0.764–0.949) | 46.4 | 93.7 | Train | |
KNN | 65.4 | 0.687 (0.489–0.886) | 41.7 | 85.7 | Test | |
Random forest | 86.7 | 0.943 (0.891–0.995) | 96.4 | 78.1 | Train | |
Random forest | 69.2 | 0.732 (0.533–0.931) | 91.7 | 50.0 | Test | |
DWI | LR | 73.3 | 0.788 (0.671–0.905) | 67.9 | 78.1 | Train |
LR | 61.5 | 0.685 (0.473–0.896) | 75.0 | 50.0 | Test | |
SVM | 81.7 | 0.848 (0.740–0.956) | 75.0 | 87.5 | Train | |
SVM | 61.5 | 0.607 (0.375–0.840) | 58.3 | 64.3 | Test | |
KNN | 66.7 | 0.808 (0.700–0.916) | 32.1 | 96.9 | Train | |
KNN | 57.7 | 0.601 (0.377–0.825) | 8.3 | 100.0 | Test | |
Random forest | 88.3 | 0.949 (0.899–0.999) | 75.0 | 100.0 | Train | |
Random forest | 65.4 | 0.565 (0.321–0.810) | 25.0 | 100.0 | Test | |
ALL | LR | 83.3 | 0.888 (0.803–0.974) | 92.9 | 75.0 | Train |
LR | 73.1 | 0.815 (0.648–0.983) | 91.7 | 57.1 | Test | |
SVM | 86.7 | 0.921 (0.849–0.992) | 92.9 | 81.2 | Train | |
SVM | 73.1 | 0.768 (0.576–0.960) | 66.7 | 78.6 | Test | |
KNN | 80.0 | 0.909 (0.844–0.974) | 82.1 | 78.1 | Train | |
KNN | 65.4 | 0.771 (0.594–0.948) | 83.3 | 50.0 | Test | |
Random forest | 86.7 | 0.967 (0.931–1.000) | 85.7 | 87.5 | Train | |
Random forest | 73.1 | 0.690 (0.460–0.921) | 58.3 | 85.7 | Test |
ALL = T1WI + T2WI + DWI. AUC, area under the curve; CI, confidence interval; DWI, diffusion-weighted imaging; KNN, k-nearest neighbors; LR, logistic regression; SVM, support vector machine; T1WI, T1-weighted imaging; T2WI, T2-weighted imaging.
Among the four models, the LR classifier demonstrated superior performance, showcasing enhanced generalization capability. Therefore, to ensure the stability and reliability of our study, we used the LR classifier as the optimal radiomics model for comparing and evaluating multisequence MRI. Furthermore, compared to that of the individual sequences, the performance of the combined sequence model was found to be superior.
Model comparison
The receiver operating characteristic (ROC) curves for the individual sequence and combined sequence LR models in both the training and test sets are illustrated in Figure 4A. Compared to the individual sequence model, the combined sequence model demonstrated an enhanced recognition capability, achieving AUC values of 0.888 [95% confidence interval (CI): 0.803–0.974] for the training set and 0.815 (95% CI: 0.648–0.983) for the test set. DCA indicated that the combined sequence model outperformed the individual sequence model, providing superior clinical net benefits across a wide range of threshold probabilities (Figure 4B). Furthermore, calibration curves indicated that the combined sequence model exhibited relatively good calibration performance (Figure 4C).
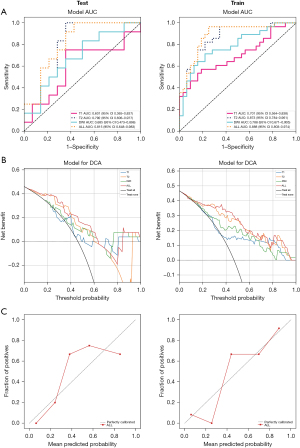
Discussion
LA-NSCLC demonstrates significant tumor heterogeneity, and radiotherapy remains a critical treatment for unresectable cases. This highlights the necessity of reliable predictive tools to distinguish between patients sensitive to tumor regression and those resistant. The ability to predict the efficacy of radiotherapy in patients with LA-NSCLC prior to treatment holds significant implications for the development of personalized therapeutic strategies. Zhou et al. (38) evaluated the physiological changes of tumors during large fraction stereotactic radiotherapy using multiparametric MRI. Their findings revealed that tumor heterogeneity could be observed on multiparametric images, further underscoring the potential and value of MRI in predicting LA-NSCLC. In recent years, with the rapid advancement of MRI technology, its application in the diagnosis, staging, and efficacy evaluation of lung cancer has garnered increased attention. Notably, the incorporation of sequences such as DWI has propelled lung cancer assessment to a level of molecular functional. Therefore, the objective of this study was to establish a predictive model using multisequence MR images obtained prior to radiotherapy that could aid in the early prediction of treatment outcomes.
Previous studies have primarily assessed the tumor response of NSCLC through the radiomics features obtained from CT and PET-CT imaging. Zhou et al. (39) developed a nomogram model based on CT images to predict the benefits of adaptive radiotherapy for patients with LA-NSCLC. They found that the tumor regression assessment model constructed using RECIST 1.1 demonstrated superior performance, with an AUC value of 0.771. Cilla et al. (40) used radiomics based on CT imaging to predict the CR in patients with lung metastases following stereotactic radiation therapy. The machine learning models constructed with LR and classification tree analysis yielded AUC values for CR prediction of 0.707 and 0.753, respectively. Ohno et al. (41) conducted a study indicating the superiority of DWI in predicting tumor response to chemotherapy and radiotherapy in patients with NSCLC. The specificity of DWI was found to be significantly higher than that of PET-CT, with values of 44.4% for DWI compared to 11.1% for PET-CT. Additionally, the accuracy of DWI (76.6%) also surpassed that of PET-CT (67.2%). Our findings are similar, with the combined sequence MRI model achieving an AUC of 0.815, an accuracy of 73.1%, and a sensitivity of 91.7% in the test set. These results demonstrate performance comparable, or even superior to, previous studies on radiomics for lung cancer using CT and PET-CT imaging modalities (42-44). However, the specificity of the joint sequence test set is 57.1%. A possible explanation for this finding is that the relatively small sample size in this study may have resulted in a test set that does not adequately represent the target population. To address this issue, we will focus on expanding the sample size and optimizing the model structure in future research. Although CT and PET-CT play a significant role in the assessment of radiotherapy efficacy for lung cancer, they have certain limitations. Several studies suggest that MRI may be valuable in evaluating treatment response under DWI sequences (45,46). In recent years, the application of MRI in the field of lung cancer has become increasingly prevalent. Its superior soft-tissue contrast allows for a clearer visualization of the invasion of lung cancer into structures such as the chest wall and mediastinum. Furthermore, MRI does not involve ionizing radiation, thereby reducing potential risks to patients (47,48).
In this study, compared to individual sequence models, the combined sequence model enhanced sensitivity by integrating information from multiple sequences, thereby reducing the incidence of missed diagnoses. Furthermore, in terms of accuracy, the combined sequence model effectively decreased the likelihood of misjudgments, making the model more accurate and reliable during application. Numerous studies have demonstrated the advantages of MRI combined sequences. Xie et al. (49) established a radiomics model to differentiate between benign and malignant solid pulmonary nodules. They found that the model based on multisequence MRI (T1WI + T2WI + apparent diffusion coefficient) exhibited the best diagnostic performance, with an AUC of 0.858 (95% CI: 0.775–0.919) for the training set and an AUC of 0.774 (95% CI: 0.621–0.887) for the validation set. This provides strong support for the early prediction of treatment outcomes. Yu et al. (50) conducted a retrospective study involving 86 patients with ovarian cancer, aiming to predict peritoneal carcinomatosis based on preoperative fat-suppressed (FS) T2WI, DWI, and dynamic contrast-enhanced MRI (DCE-MRI) sequences. The results indicated that the AUC of the combined imaging model was superior to that of the individual models for FS-T2WI, DWI, and DCE-MRI (with respective values of 0.846, 0.762, 0.830, and 0.807). However, there is currently a lack of research in the field related to LA-NSCLC. The novelty of this study lies in the innovative use of MRI radiomics to assess tumor response following radiotherapy in patients with LA-NSCLC, demonstrating the advantages and potential of a combined-sequence MRI radiomics model in lung cancer evaluation. It addresses a significant deficiency in this area, not only broadening the application scope of radiomics in assessing radiotherapy efficacy but also providing new perspectives for personalized treatment strategies in lung cancer radiotherapy in the future. The clinical decision curve indicates that our combined sequence model can enhance the treatment decision-making process at relatively high thresholds, demonstrating improved clinical applicability (51).
This study also involved several limitations: (I) we employed a single-institution design with a relatively small sample size, necessitating larger sample sizes and external validation cohorts to enhance the reliability of the model. (II) Compared to individual-sequence radiomics models, combined sequence models demand higher data processing and computational resources. Therefore, in future work, we will further employ additional machine learning algorithms and computational strategies to improve efficiency. (III) Our research focused solely on primary tumors; in the future, we may conduct multiregional radiomic analyses on metastatic lymph nodes or the entire lung to further explore the potential value of MRI radiomics in the field of lung cancer.
Conclusions
The establishment of a model to predict tumor response to radiotherapy in patients with LA-NSCLC using multi-sequence MRI radiomic features is feasible. Among the various models, the combined sequence model demonstrates relatively superior predictive performance and clinical applicability, potentially paving new avenues for precision treatment in patients with LA-NSCLC.
Acknowledgments
We gratefully acknowledge the financial support provided by the Haiyan Fund of the Harbin Medical University Cancer Hospital (No. JJMS 2023-15).
Footnote
Reporting Checklist: The authors have completed the TRIPOD reporting checklist. Available at https://jtd.amegroups.com/article/view/10.21037/jtd-2025-142/rc
Data Sharing Statement: Available at https://jtd.amegroups.com/article/view/10.21037/jtd-2025-142/dss
Peer Review File: Available at https://jtd.amegroups.com/article/view/10.21037/jtd-2025-142/prf
Funding: This study was supported by
Conflicts of Interest: All authors have completed the ICMJE uniform disclosure form (available at https://jtd.amegroups.com/article/view/10.21037/jtd-2025-142/coif). J.X. reports funding support from the Haiyan Fund of the Harbin Medical University Cancer Hospital (No. JJMS 2023-15). B.A. receives research grant from MSDAvenir, consulting fees from Novartis, Astellas, Sanofi, payment for lectures from Sanofi, AstraZeneca, BMS, MSD, Astellas, and support for attending meetings from Janssen, MSD, Pfizer, IPSEN Pharma, Takeda. The other authors have no conflicts of interest to declare.
Ethical Statement: The authors are accountable for all aspects of the work in ensuring that questions related to the accuracy or integrity of any part of the work are appropriately investigated and resolved. The study was conducted in accordance with the Declaration of Helsinki (as revised in 2013). The study was approved by the Ethics Committee of Harbin Medical University Cancer Hospital (No. KY2023-05) and individual consent for this retrospective analysis was waived.
Open Access Statement: This is an Open Access article distributed in accordance with the Creative Commons Attribution-NonCommercial-NoDerivs 4.0 International License (CC BY-NC-ND 4.0), which permits the non-commercial replication and distribution of the article with the strict proviso that no changes or edits are made and the original work is properly cited (including links to both the formal publication through the relevant DOI and the license). See: https://creativecommons.org/licenses/by-nc-nd/4.0/.
References
- Bray F, Laversanne M, Sung H, et al. Global cancer statistics 2022: GLOBOCAN estimates of incidence and mortality worldwide for 36 cancers in 185 countries. CA Cancer J Clin 2024;74:229-63.
- Kim HC, Ji W, Lee JC, et al. Prognostic Factor and Clinical Outcome in Stage III Non-Small Cell Lung Cancer: A Study Based on Real-World Clinical Data in the Korean Population. Cancer Res Treat 2021;53:1033-41.
- Tuminello S, Untalan M, Patel K, et al. Methodological aspects of studies on survival after immunotherapy in stage IV non-small cell lung cancer. Transl Lung Cancer Res 2025;14:303-4.
- Carroll NM, Eisenstein J, Freml JM, et al. Association of systemic therapy with survival among adults with advanced non-small cell lung cancer. Transl Lung Cancer Res 2025;14:176-93.
- Wu J, Ni T, Deng R, et al. Safety and efficacy of radiotherapy/chemoradiotherapy combined with immune checkpoint inhibitors for non-small cell lung cancer: A systematic review and meta-analysis. Front Immunol 2023;14:1065510.
- Grambozov B, Stana M, Zehentmayr F. Immediate start of durvalumab after chemoradiotherapy in unresectable non-small cell lung cancer UICC stage III: early results from the TORG1937/DATE study. J Thorac Dis 2025;17:1-4.
- Faivre-Finn C, Vicente D, Kurata T, et al. Four-Year Survival With Durvalumab After Chemoradiotherapy in Stage III NSCLC-an Update From the PACIFIC Trial. J Thorac Oncol 2021;16:860-7.
- Aupérin A, Le Péchoux C, Rolland E, et al. Meta-analysis of concomitant versus sequential radiochemotherapy in locally advanced non-small-cell lung cancer. J Clin Oncol 2010;28:2181-90.
- Yegya-Raman N, Zou W, Nie K, et al. Advanced radiation techniques for locally advanced non-small cell lung cancer: intensity-modulated radiation therapy and proton therapy. J Thorac Dis 2018;10:S2474-91.
- Laine AM, Westover KD, Choy H. Radiation therapy as a backbone of treatment of locally advanced non-small cell lung cancer. Semin Oncol 2014;41:57-68.
- Liu D, Benzaquen J, Morris LGT, et al. Mutations in KMT2C, BCOR and KDM5C Predict Response to Immune Checkpoint Blockade Therapy in Non-Small Cell Lung Cancer. Cancers (Basel) 2022;14:2816.
- Yin X, Liao H, Yun H, et al. Artificial intelligence-based prediction of clinical outcome in immunotherapy and targeted therapy of lung cancer. Semin Cancer Biol 2022;86:146-59.
- de Kock R, Borne BVD, Soud MY, et al. Circulating biomarkers for monitoring therapy response and detection of disease progression in lung cancer patients. Cancer Treat Res Commun 2021;28:100410.
- Shields MD, Chen K, Dutcher G, et al. Making the Rounds: Exploring the Role of Circulating Tumor DNA (ctDNA) in Non-Small Cell Lung Cancer. Int J Mol Sci 2022;23:9006.
- Lambin P, Rios-Velazquez E, Leijenaar R, et al. Radiomics: extracting more information from medical images using advanced feature analysis. Eur J Cancer 2012;48:441-6.
- Mattonen SA, Huang K, Ward AD, et al. New techniques for assessing response after hypofractionated radiotherapy for lung cancer. J Thorac Dis 2014;6:375-86.
- Shi L, He Y, Yuan Z, et al. Radiomics for Response and Outcome Assessment for Non-Small Cell Lung Cancer. Technol Cancer Res Treat 2018;17:1533033818782788.
- Chen B, Yang L, Zhang R, et al. Radiomics: an overview in lung cancer management—a narrative review. Ann Transl Med 2020;8:1191.
- Konert T, Everitt S, La Fontaine MD, et al. Robust, independent and relevant prognostic 18F-fluorodeoxyglucose positron emission tomography radiomics features in non-small cell lung cancer: Are there any? PLoS One 2020;15:e0228793.
- van Timmeren JE, van Elmpt W, Leijenaar RTH, et al. Longitudinal radiomics of cone-beam CT images from non-small cell lung cancer patients: Evaluation of the added prognostic value for overall survival and locoregional recurrence. Radiother Oncol 2019;136:78-85.
- Wang D, Qiu B, He H, et al. Tumor response evaluation by combined modalities of chest magnetic resonance imaging and computed tomography in locally advanced non-small cell lung cancer after concurrent chemoradiotherapy. Radiother Oncol 2022;168:211-20.
- Zhang H, Fu C, Fan M, et al. Reduction of inter-observer variability using MRI and CT fusion in delineating of primary tumor for radiotherapy in lung cancer with atelectasis. Front Oncol 2022;12:841771.
- Mahdavi Rashed M, Nekooei S, Nouri M, et al. Evaluation of DWI and ADC Sequences' Diagnostic Values in Benign and Malignant Pulmonary Lesions. Turk Thorac J 2020;21:390-6.
- Stadlbauer A, Marhold F, Oberndorfer S, et al. Metabolic Tumor Microenvironment Characterization of Contrast Enhancing Brain Tumors Using Physiologic MRI. Metabolites 2021;11:668.
- Bennani-Baiti B, Pinker K, Zimmermann M, et al. Non-Invasive Assessment of Hypoxia and Neovascularization with MRI for Identification of Aggressive Breast Cancer. Cancers (Basel) 2020;12:2024.
- Jagoda P, Fleckenstein J, Sonnhoff M, et al. Diffusion-weighted MRI improves response assessment after definitive radiotherapy in patients with NSCLC. Cancer Imaging 2021;21:15.
- Kumar S, Liney G, Rai R, et al. Magnetic resonance imaging in lung: a review of its potential for radiotherapy. Br J Radiol 2016;89:20150431.
- Meier-Schroers M, Homsi R, Skowasch D, et al. Lung cancer screening with MRI: results of the first screening round. J Cancer Res Clin Oncol 2018;144:117-25.
- Goldstraw P, Chansky K, Crowley J, et al. The IASLC Lung Cancer Staging Project: Proposals for Revision of the TNM Stage Groupings in the Forthcoming (Eighth) Edition of the TNM Classification for Lung Cancer. J Thorac Oncol 2016;11:39-51.
- Lalchandani UR, Sahai V, Hersberger K, et al. A Radiologist's Guide to Response Evaluation Criteria in Solid Tumors. Curr Probl Diagn Radiol 2019;48:576-85.
- Yang G, Bai J, Hao M, et al. Enhancing recurrence risk prediction for bladder cancer using multi-sequence MRI radiomics. Insights Imaging 2024;15:88.
- Li Y, Lv X, Wang B, et al. Predicting EGFR T790M Mutation in Brain Metastases Using Multisequence MRI-Based Radiomics Signature. Acad Radiol 2023;30:1887-95.
- Peiliang Wang MD, Yikun Li MM, Mengyu Zhao MM, et al. Distinguishing immune checkpoint inhibitor-related pneumonitis from radiation pneumonitis by CT radiomics features in non-small cell lung cancer. Int Immunopharmacol 2024;128:111489.
- Hu D, Li X, Lin C, et al. Deep Learning to Predict the Cell Proliferation and Prognosis of Non-Small Cell Lung Cancer Based on FDG-PET/CT Images. Diagnostics (Basel) 2023;13:3107.
- Wang J, Chen J, Zhou R, et al. Machine learning-based multiparametric MRI radiomics for predicting poor responders after neoadjuvant chemoradiotherapy in rectal Cancer patients. BMC Cancer 2022;22:420.
- Wu M, Xu W, Fei Y, et al. MRI-based clinical radiomics nomogram may predict the early response after concurrent chemoradiotherapy in locally advanced nasopharyngeal carcinoma. Front Oncol 2023;13:1192953.
- Fan Y, Dong Y, Wang H, et al. Development and externally validate MRI-based nomogram to assess EGFR and T790M mutations in patients with metastatic lung adenocarcinoma. Eur Radiol 2022;32:6739-51.
- Zhou H, Zhang Z, Denney R, et al. Tumor physiological changes during hypofractionated stereotactic body radiation therapy assessed using multi-parametric magnetic resonance imaging. Oncotarget 2017;8:37464-77.
- Zhou C, Hou L, Tang X, et al. CT-based radiomics nomogram may predict who can benefit from adaptive radiotherapy in patients with local advanced-NSCLC patients. Radiother Oncol 2023;183:109637.
- Cilla S, Pistilli D, Romano C, et al. CT-based radiomics prediction of complete response after stereotactic body radiation therapy for patients with lung metastases. Strahlenther Onkol 2023;199:676-85.
- Ohno Y, Koyama H, Yoshikawa T, et al. Diffusion-weighted MRI versus 18F-FDG PET/CT: performance as predictors of tumor treatment response and patient survival in patients with non-small cell lung cancer receiving chemoradiotherapy. AJR Am J Roentgenol 2012;198:75-82.
- Nakajo M, Jinguji M, Ito S, et al. Clinical application of (18)F-fluorodeoxyglucose positron emission tomography/computed tomography radiomics-based machine learning analyses in the field of oncology. Jpn J Radiol 2024;42:28-55.
- Kothari G, Korte J, Lehrer EJ, et al. A systematic review and meta-analysis of the prognostic value of radiomics based models in non-small cell lung cancer treated with curative radiotherapy. Radiother Oncol 2021;155:188-203.
- Walls GM, Osman SOS, Brown KH, et al. Radiomics for Predicting Lung Cancer Outcomes Following Radiotherapy: A Systematic Review. Clin Oncol (R Coll Radiol) 2022;34:e107-22.
- Bak SH, Kim C, Kim CH, et al. Magnetic resonance imaging for lung cancer: a state-of-the-art review. Precision and Future Medicine 2022;6:49-77.
- Campbell-Washburn AE, Ramasawmy R, Restivo MC, et al. Opportunities in Interventional and Diagnostic Imaging by Using High-Performance Low-Field-Strength MRI. Radiology 2019;293:384-93.
- Ciliberto M, Kishida Y, Seki S, et al. Update of MR Imaging for Evaluation of Lung Cancer. Radiol Clin North Am 2018;56:437-69.
- Sommer G, Tremper J, Koenigkam-Santos M, et al. Lung nodule detection in a high-risk population: comparison of magnetic resonance imaging and low-dose computed tomography. Eur J Radiol 2014;83:600-5.
- Xie K, Cui C, Li X, et al. MRI-Based Clinical-Imaging-Radiomics Nomogram Model for Discriminating Between Benign and Malignant Solid Pulmonary Nodules or Masses. Acad Radiol 2024;31:4231-41.
- Yu XY, Ren J, Jia Y, et al. Multiparameter MRI Radiomics Model Predicts Preoperative Peritoneal Carcinomatosis in Ovarian Cancer. Front Oncol 2021;11:765652.
- Huang YQ, Liang CH, He L, et al. Development and Validation of a Radiomics Nomogram for Preoperative Prediction of Lymph Node Metastasis in Colorectal Cancer. J Clin Oncol 2016;34:2157-64.